Weekly Reading List: Dec 23, 2024
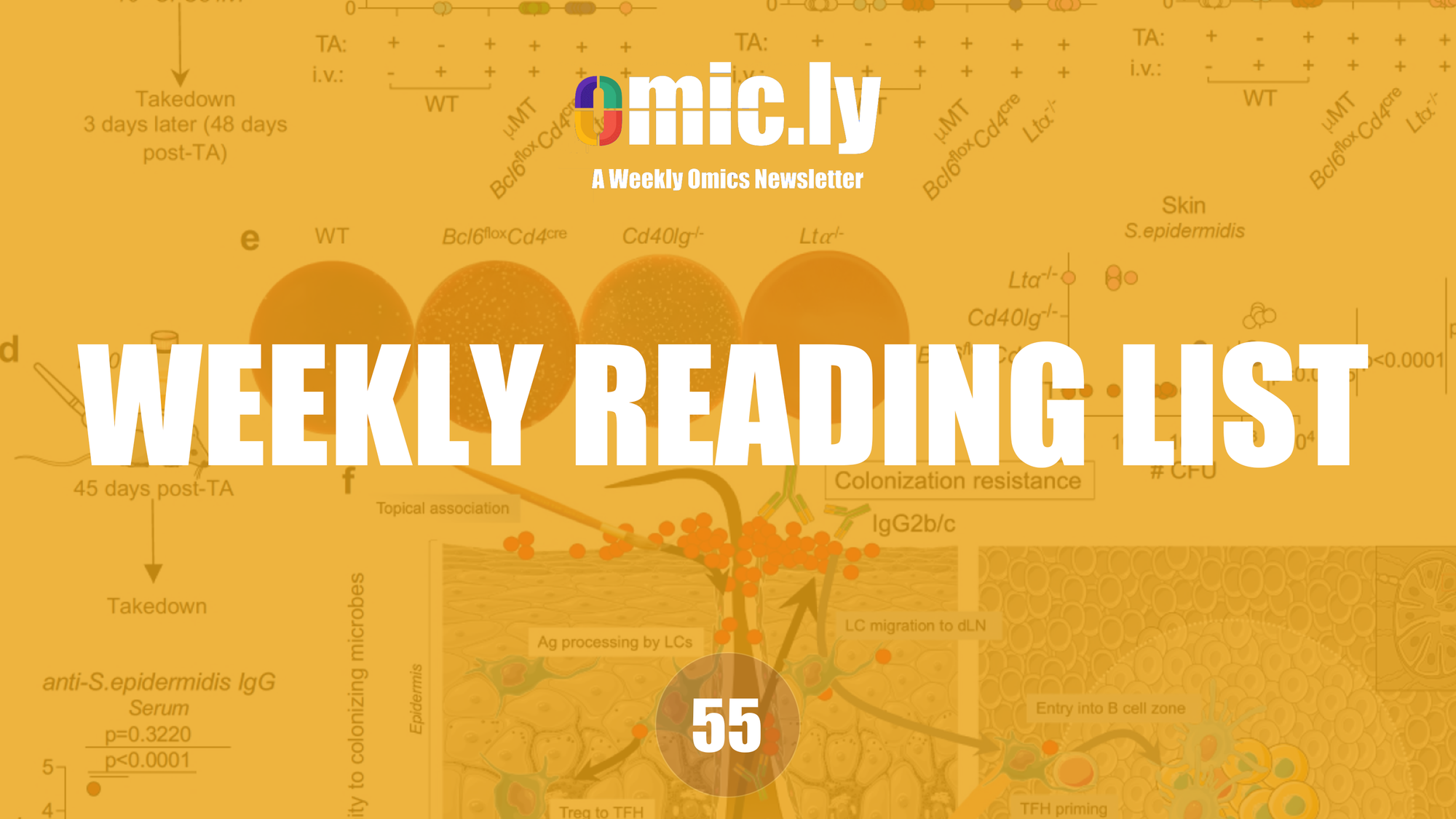
CRISPR genome-editing grows up: advanced therapies head for the clinic
Gene-editing technologies for cancer and blood disorders are maturing a little more than a year after the first CRISPR drug was approved.
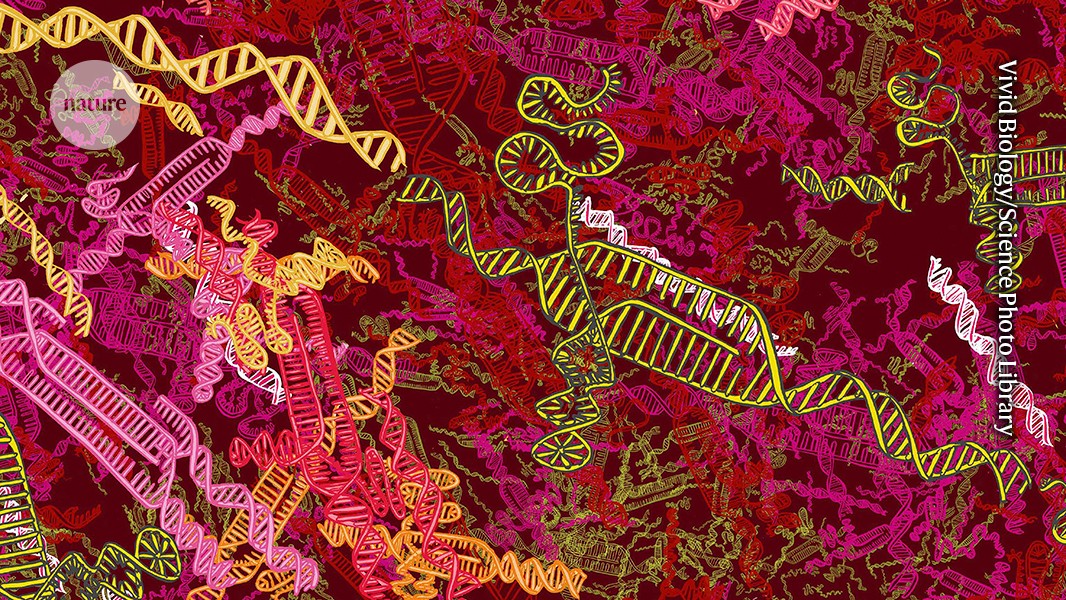
Study Failure Spotlights Challenges for ctDNA Strategies in Breast Cancer Drug Trials
Researchers stopped a Phase III trial testing GlaxoSmithKline's PARP inhibitor Zejula (niraparib) as an adjuvant treatment of early-stage breast cancer due to low detection of circulating tumor DNA (ctDNA) in patients after their initial treatment, investigators said Friday at the San Antonio Breast Cancer Symposium.
Fierce Biotech’s Rotten Tomatoes of 2024
Biotech experienced a year of mixed fortunes in 2024. | Fierce Biotech recounts the biggest biotech blunders and one inspiring intervention in the 2024 edition of Rotten Tomatoes.
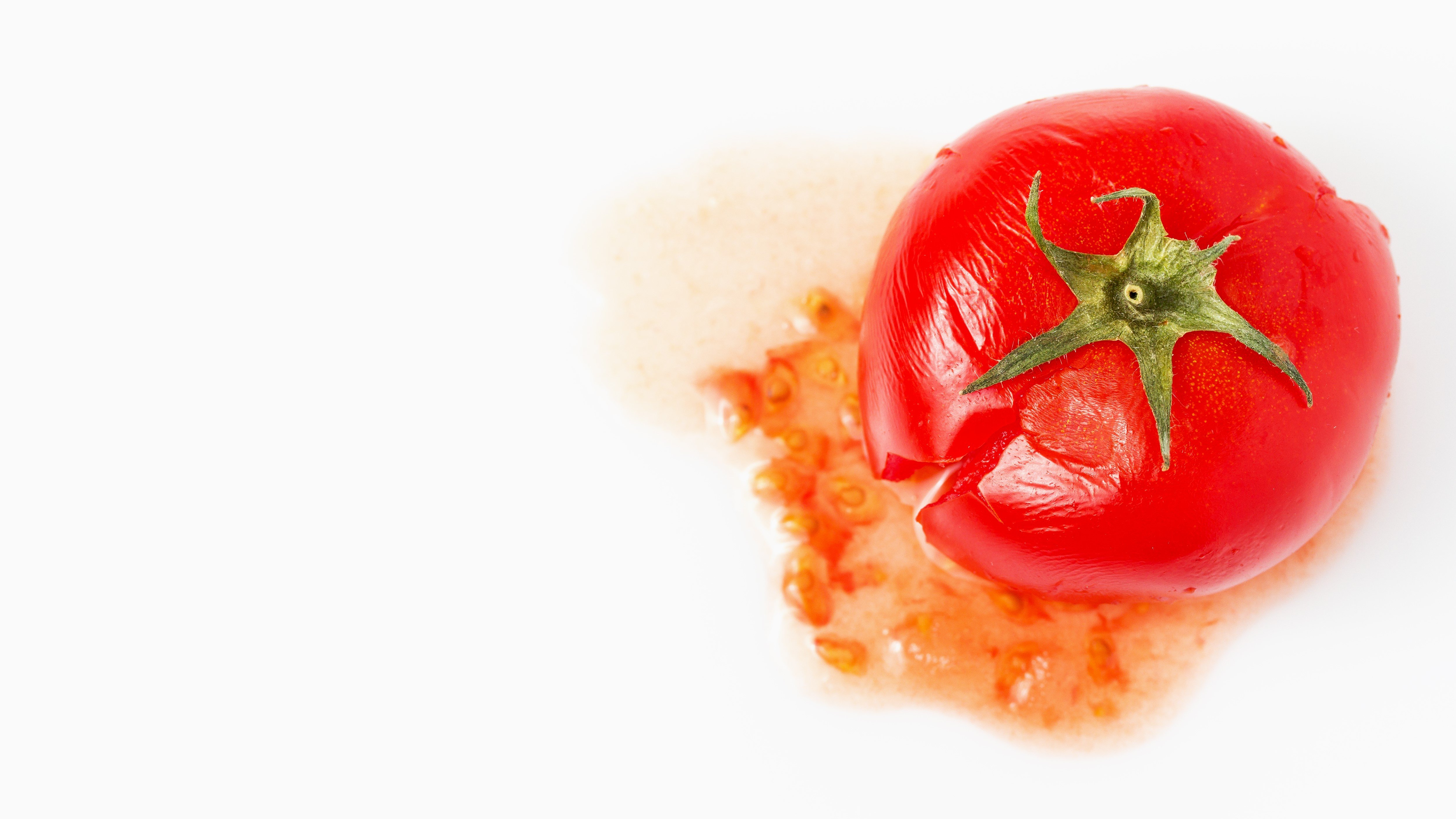
From early methods for DNA diagnostics to genomes and epigenomes at high resolution during four decades – a personal perspective | Upsala Journal of Medical Sciences

Structural basis of H3K36 trimethylation by SETD2 during chromatin transcription
During transcription, RNA polymerase II traverses through chromatin, and post-translational modifications including histone methylations mark regions of active transcription. Histone protein H3 lysine 36 trimethylation (H3K36me3), which is established by the histone methyltransferase SETD2, suppresses cryptic transcription, regulates splicing, and serves as a binding site for transcription elongation factors.
Metabolic rearrangement enables adaptation of microbial growth rate to temperature shifts - Nature Microbiology
Growth rate dynamics after temperature shifts can be explained by metabolic rearrangement due to temperature-sensitive enzyme activities in bacteria and yeast.
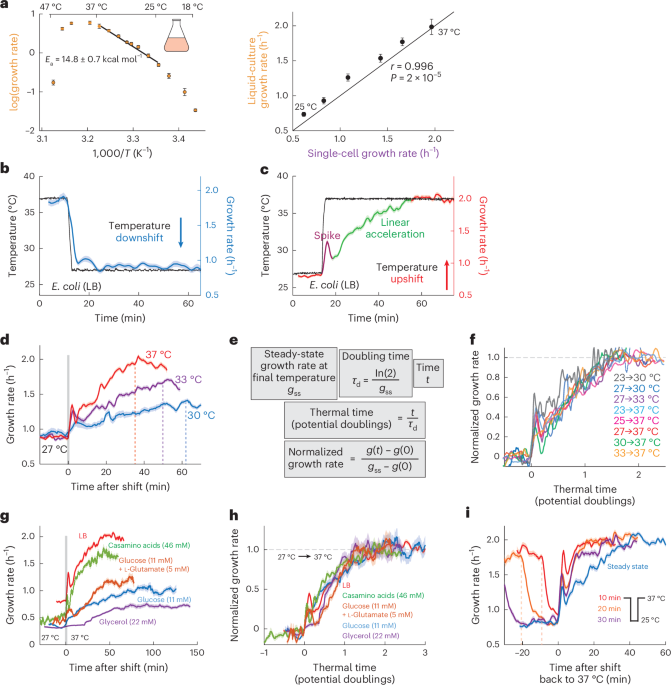
Keeping it in the family: using protein family templates to rescue low confidence AlphaFold2 models
High confidence structure prediction models have become available for nearly all protein sequences. More than 200 million AlphaFold2 models are now publicly available. We observe that there can be significant variability in the prediction confidence as judged by plDDT scores across a protein family. We have explored whether the predictions with lower plDDT in a family can be improved by the use of higher plDDT templates from the family as template structures in AlphaFold2.
The Causal Pivot: A Structural Approach to Genetic Heterogeneity and Variant Discovery in Complex Diseases
We present the Causal Pivot (CP) as a structural causal model (SCM) for analyzing genetic heterogeneity in complex diseases. The CP leverages one established causal factor to detect the contribution of a second suspected cause. Specifically, polygenic risk scores (PRS) serve as known causes, while rare variants (RV) or RV ensembles are evaluated as candidate causes. The CP incorporates outcome-induced association by conditioning on disease status. We derive a conditional maximum likelihood procedure for binary and quantitative traits and develop the Causal Pivot Likelihood Ratio Test (CP-LRT) to detect causal signals. Through simulations, we demonstrate the CP-LRT’s robust power and superior error control compared to alternatives. We apply the CP-LRT to UK Biobank (UKB) data, analyzing three exemplar diseases: hypercholesterolemia (HC, LDL-c ≥ 4.9 mmol/L; nc=24,656), breast cancer (BC, ICD10 C50; nc=12,479), and Parkinson’s disease (PD, ICD10 G20; nc=2,940). For PRS, we utilize UKB-derived values, and for RVs, we analyze ClinVar pathogenic/likely pathogenic variants and loss-of-function mutations in disease-relevant genes: LDLR for HC, BRCA1 for BC, and GBA for PD. Significant CP-LRT signals were detected for all three diseases. Cross-disease and synonymous variant analyses serve as controls. We further develop ancestry adjustment using matching and inverse probability weighting, and we extend the CP to examine oligogenic burden in the lysosomal storage pathway for PD. The CP reveals an approach to address heterogeneity and is an extensible method for inference and discovery in complex disease genetics. ### Competing Interest Statement The authors have declared no competing interest.
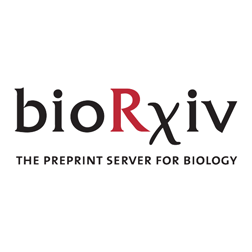
Bridging genomics’ greatest challenge: The diversity gap
Achieving diverse representation in biomedical data is critical for healthcare equity. Failure to do so perpetuates health disparities and exacerbates biases that may harm patients with underrepresented ancestral backgrounds. We present a quantitative assessment of representation in datasets used across human genomics, including genome-wide association studies (GWASs), pharmacogenomics, clinical trials, and direct-to-consumer (DTC) genetic testing. We suggest that relative proportions of ancestries represented in datasets, compared to the global census population, provide insufficient representation of global ancestral genetic diversity.
She took a DNA test for fun. Police used it to charge her grandmother with murder in a cold case
By Taylor Galgano(CNN) — It was the middle of Jenna Gerwatowski’s workday at the local flower shop in Newberry, Michigan, when she got a call from an unknown number.The now 23-year-old doesn’t u
Specificity, length, and luck: How genes are prioritized by rare and common variant association studies
Standard genome-wide association studies (GWAS) and rare variant burden tests are essential tools for identifying trait-relevant genes. Although these methods are conceptually similar, we show by analyzing association studies of 209 quantitative traits in the UK Biobank that they systematically prioritize different genes. This raises the question of how genes should ideally be prioritized. We propose two prioritization criteria: 1) trait importance — how much a gene quantitatively affects a trait; and 2) trait specificity — a gene’s importance for the trait under study relative to its importance across all traits. We find that GWAS prioritize genes near trait-specific variants , while burden tests prioritize trait-specific genes . Because non-coding variants can be context specific, GWAS can prioritize highly pleiotropic genes, while burden tests generally cannot. Both study designs are also affected by distinct trait-irrelevant factors, complicating their interpretation. Our results illustrate that burden tests and GWAS reveal different aspects of trait biology and suggest ways to improve their interpretation and usage. ### Competing Interest Statement The authors have declared no competing interest.
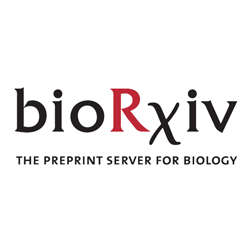
New insights into protein–protein interaction modulators in drug discovery and therapeutic advance - Signal Transduction and Targeted Therapy
Signal Transduction and Targeted Therapy - New insights into protein–protein interaction modulators in drug discovery and therapeutic advance
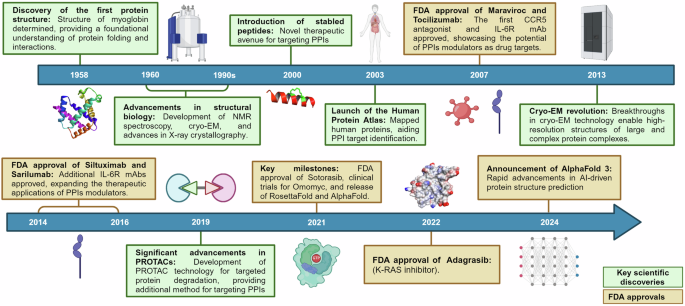
An amino acid-resolution interactome for motile cilia identifies the structure and function of ciliopathy protein complexes
Motile cilia are ancient, evolutionarily conserved organelles whose dysfunction underlies motile ciliopathies, a broad class of human diseases. Motile cilia contain a myriad of different proteins that assemble into an array of distinct machines, and understanding the interactions and functional hierarchies among them presents an important challenge. Here, we defined the protein interactome of motile axonemes using cross-linking mass spectrometry in Tetrahymena thermophila.
Gene-edited pig kidney transplanted into a third person, moving xenotransplants closer to trials
NYU Langone Health announced that, for the third time, a genetically modified pig kidney had been transplanted into a living patient
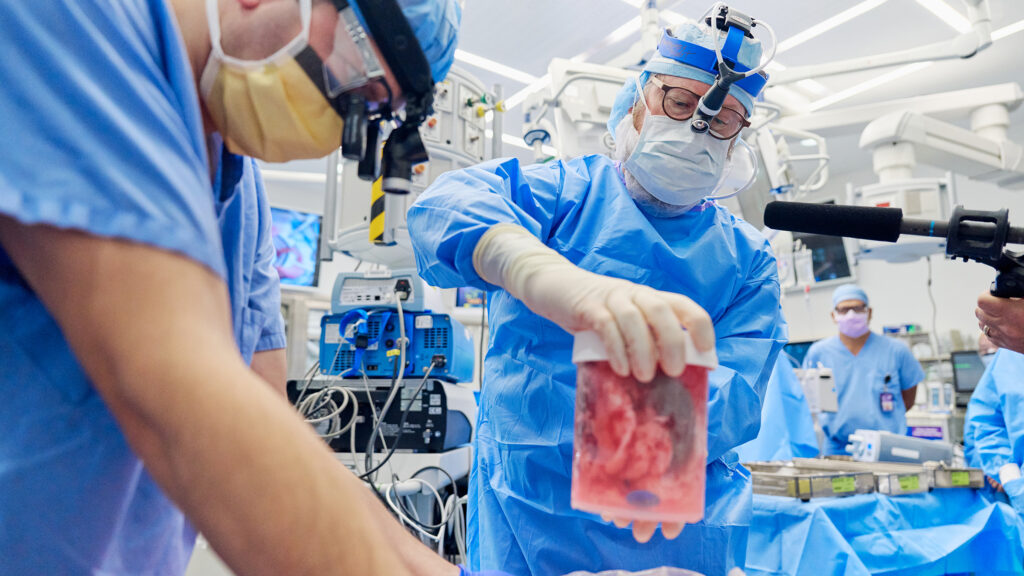
Infamous paper that popularized unproven COVID-19 treatment finally retracted
Study on hydroxychloroquine by Didier Raoult and colleagues gets pulled on ethical and scientific grounds
Roche transforms mass spectrometry diagnostics with launch of cobas® Mass Spec solution
Roche launches its cobas® Mass Spec solution, bringing mass spectrometry to the routine clinical labClinical mass spectrometry testing offers unparalleled sensitivity and specificity, providing…
The ternary complex of Mn2+, synthetic decapeptide DP1 (DEHGTAVMLK), and orthophosphate is a superb antioxidant
This study reveals the mechanism for protein radioprotection by the superb Mn-based MDP antioxidant containing Pi and decapeptide DP1, designed through considerations of Deinococcus radiodurans low molecular-weight Mn-antioxidants: The exceptionally active antioxidant in MDP is shown to be a Mn2+ (Pi/DP1) ternary complex, which, protects enzymes from radiation doses far exceeding the capabilities of Mn2+-Pi complexes alone.
LDT Final Rule Series: Part 3 – Legal Challenges
This year, we have seen several monumental events that already are, or potentially could be, pivotal to the future of the Laboratory Developed Test (“LDT”) industry – first, the issuance of the U.S.
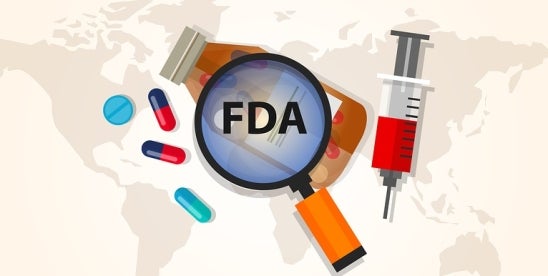
Vertex ‘ends the year in pain’ as latest non-opioid drug data disappoint
Mixed results from a study focused on lower back pain left analysts wanting as well as confused about Vertex’s plans to forge ahead in the indication.
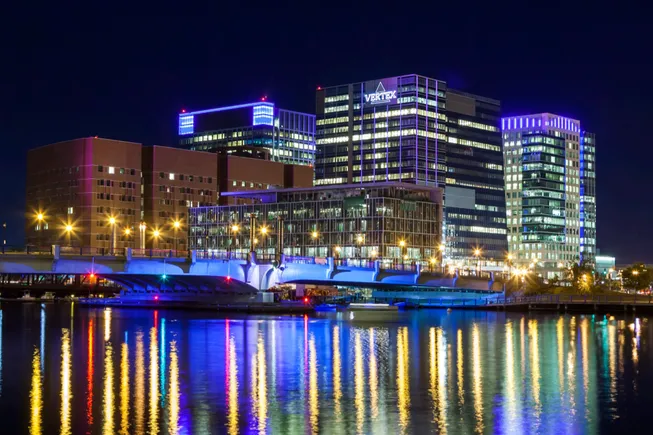
Clinical Decision Support Software Frequently Asked Questions (FAQs)
This graphic provides a visual overview of certain policies described in the Clinical Decision Support Software guidance.
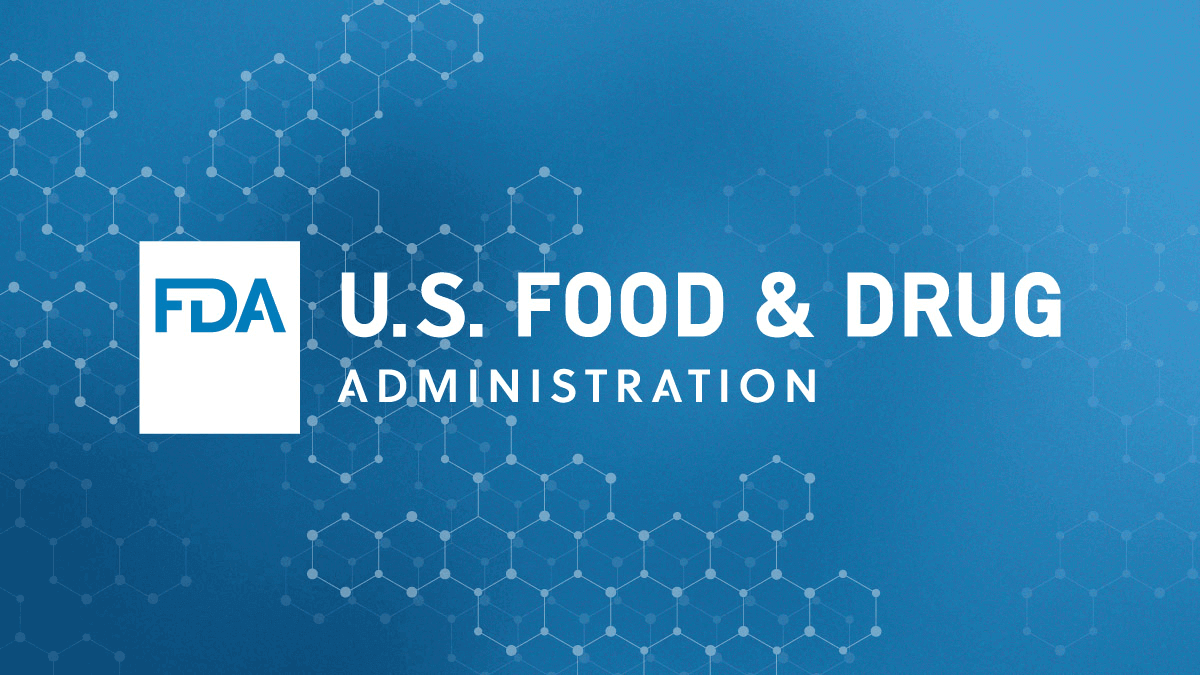
CDC confirms first severe H5N1 case in US patient
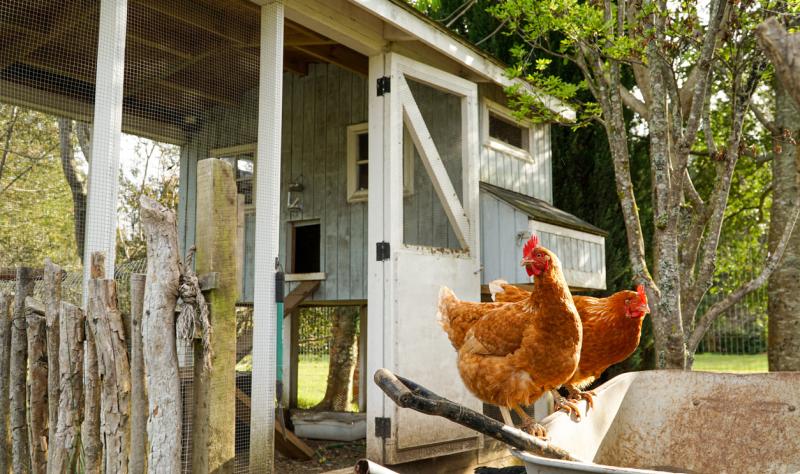
JCI -
Nicotinamide and pyridoxine stimulate muscle stem cell expansion and enhance regenerative capacity during aging

Pathway enrichment-based unsupervised learning identifies novel subtypes of cancer-associated fibroblasts in pancreatic ductal carcinoma
Existing single-cell clustering methods are based on gene expressions that are susceptible to dropout events in single-cell RNA sequencing (scRNA-seq) data. To overcome this limitation, we proposed a pathway-based clustering method for single cells (scPathClus). scPathClus first transforms single-cell gene expression matrix into pathway enrichment matrix and generates its latent feature matrix. Based on the latent feature matrix, scPathClus clusters single cells using the method of community detection. Applying scPathClus to PDAC scRNA-seq datasets, we identified two types of cancer-associated fibroblasts (CAFs), termed csCAFs and gapCAFs, which highly expressed complement system and gap junction-related pathways, respectively. Spatial transcriptome analysis revealed that gapCAFs and csCAFs are located at cancer and non-cancer regions, respectively. Pseudotime analysis suggest a potential differentiation trajectory from csCAFs to gapCAFs. Bulk transcriptome analysis showed that gapCAFs-enriched tumors are more endowed with tumor-promoting characteristics and worse clinical outcomes, while csCAFs-enriched tumors confront stronger antitumor immune responses. Compared to established CAF subtyping methods, this method displays better prognostic relevance. ### Competing Interest Statement The authors have declared no competing interest.
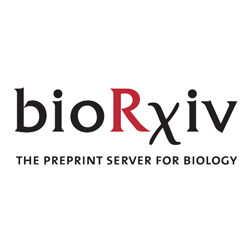