Son of a Bias, why does my sequencing dataset look like trash?
High throughput sequencing: Biases and how they can get the best of you and your data
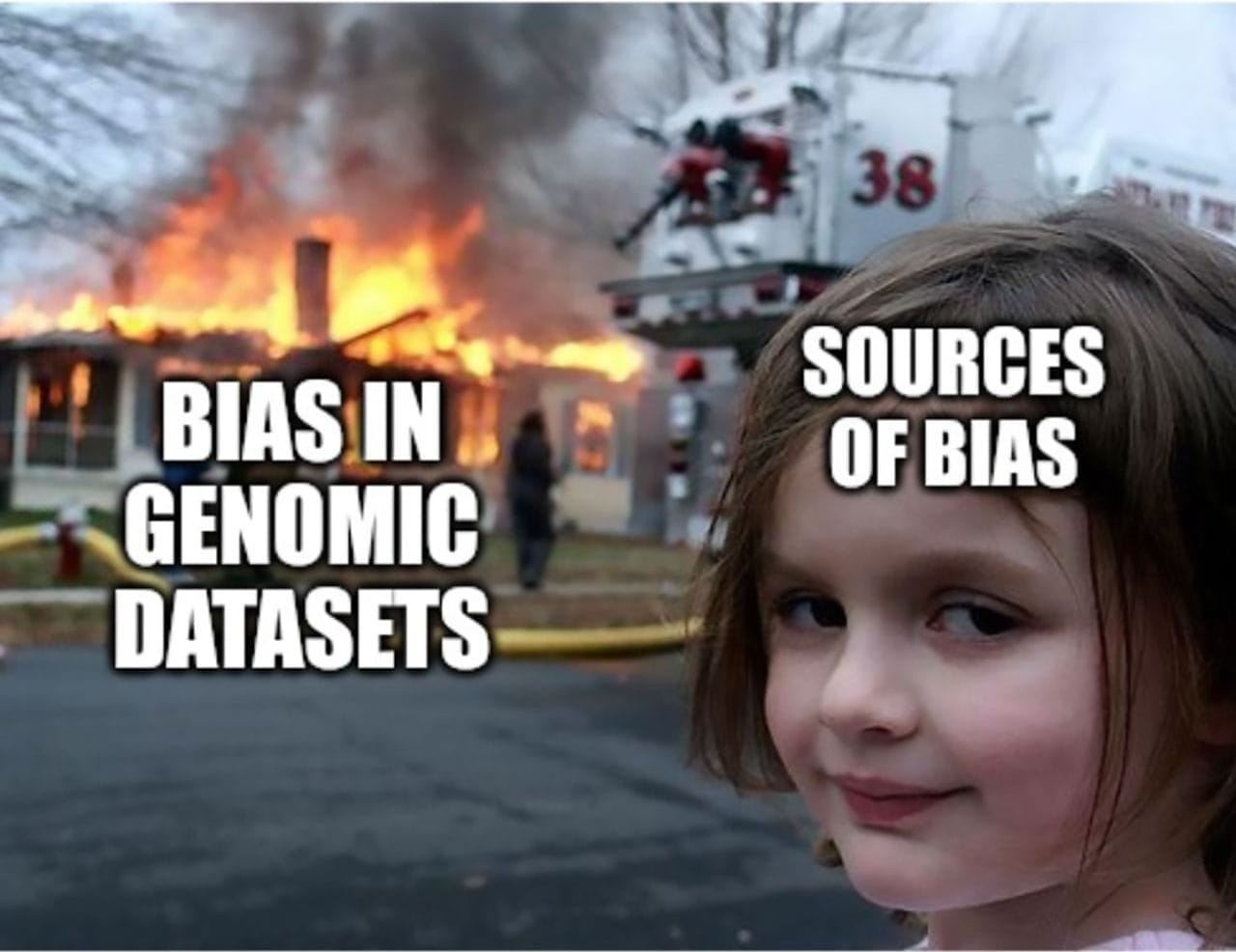
One thing that has always kept me up at night is thinking about all of the different ways that bias can be introduced into the genetic testing process.
Nearly every step presents multiple opportunities for the results to be inadvertently altered.
Bias is especially problematic in diagnostic testing and an inordinate amount of effort is expended to reduce or eliminate these biases in every step of the process.
This is because getting an answer wrong, or missing a diagnosis, has an immediate negative impact on a patient.
So what are some important sources of bias in genetic testing?
Sample Collection - This one can be tricky, especially in oncology and infectious disease because getting 'enough' or sampling the right location or region of the tissue can mean the difference between detecting the disease or infection or missing it completely. In the case of germline genetics, blood is the best and least biased source, while oral swabs and spit are potentially the most biased since your mouth is full of bacteria and, depending on the test method, this can reduce the overall quality or coverage of the final result.
Shipping/Transport - Making sure the sample gets to the testing site without degrading is a big deal. This is usually accomplished by keeping the samples cold or stabilizing them in a solution that prevents degradation of the DNA or RNA in the sample.
Nucleic Acid Extraction - The process in which you liberate the nucleic acid from cells can be a significant contributor to bias. Not all cells behave entirely the same way and this is especially true when extracting nucleic acid from bacteria, some of whom have a hard candy shell that requires a little extra oomph to be sure you get it all out in an unbiased way.
Fragmentation/Library Preparation - There are many 'easy' or 'rapid' kits on the market that introduce a substantial amount of bias into your libraries. It is important to understand the benefits and limitations of the enzymes used in each of the available methods.
Target Capture - Probe hybridization and capture is an inherently biased process because the efficiency of binding and capture is dependent on the sequence content of the region being targeted.
GCbias - This occurs as a result of PCR amplification during the library process. GC base pairs have 3 hydrogen bonds while AT pairs only have 2. PCR is biased against regions with high GC because it takes more time to push apart 3 bonds. One thing that is rarely talked about is how much less biased single molecule methods (long-reads) are than cluster based methods (short-reads). Clustering is essentially an amplification step and so will always suffer some level of bias in high GC regions.
Reference Genome/Variant Databases - Most cater to a European ancestry. This currently makes it somewhat challenging to make informed decisions in non-white populations.
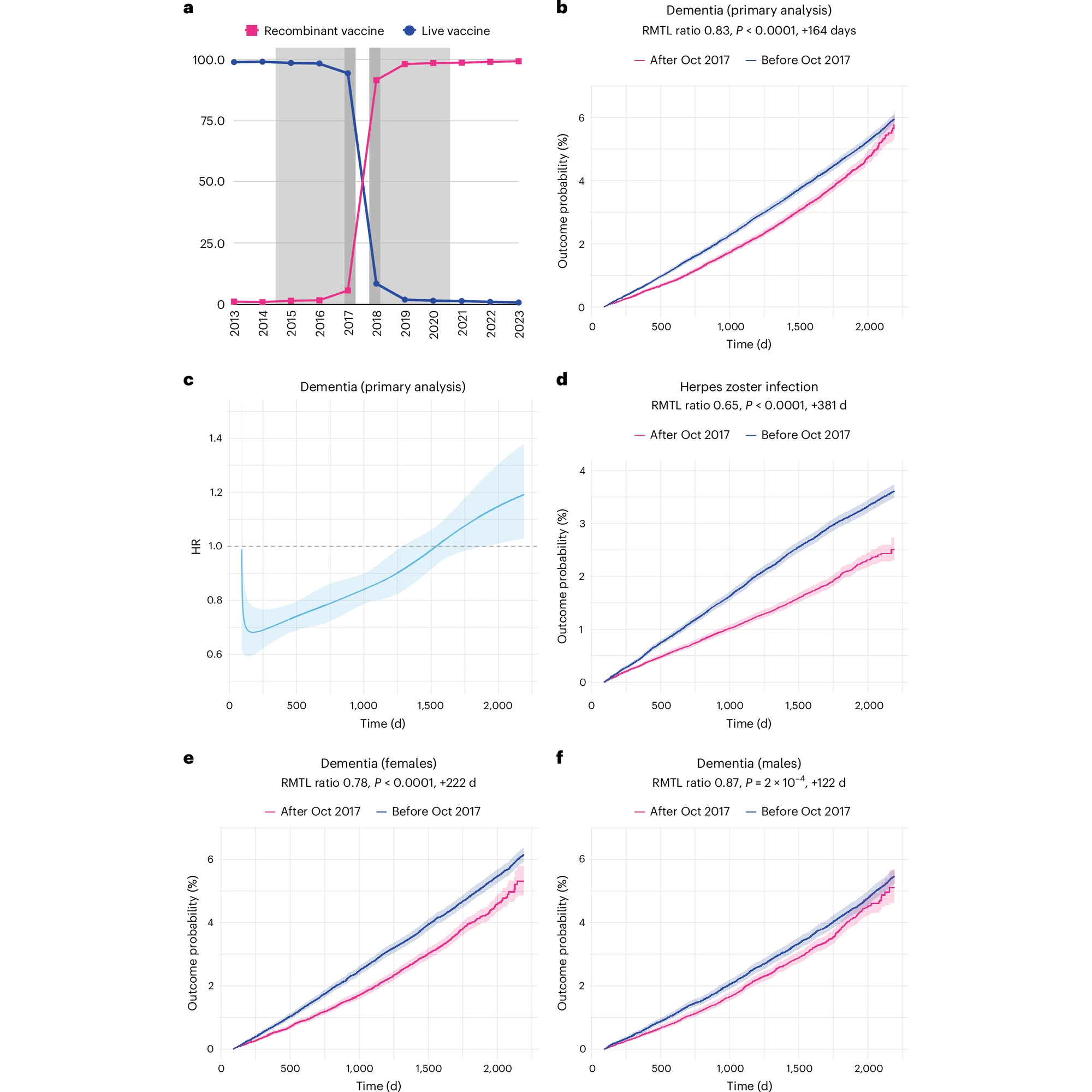