Q-scores, what they mean, and why sometimes bigger isn't necessary
High throughput sequencing metrics: Q-scores, what are they good for?
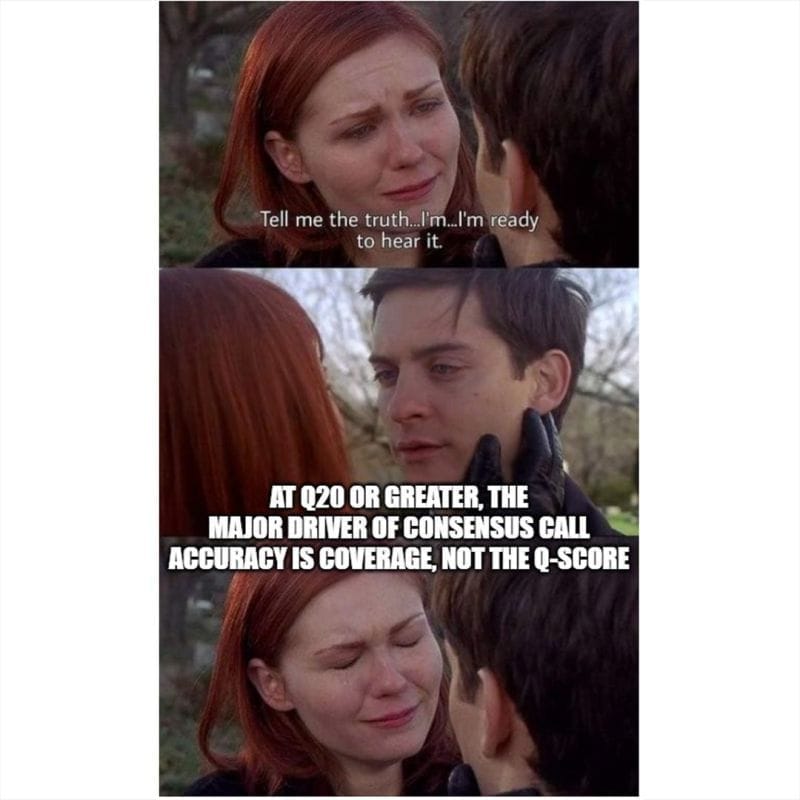
If you've done sequencing in the last 30 years, you've heard of a Q-Score and you probably know that they're a measure of quality.
But what you might not know is where this metric came from.
The idea of base quality scores emerged in the early 1990's as a result of the automation of Sanger sequencing.
These new systems used fluorescent dye terminators instead of radioactively labeled ones.
This meant that instead of looking at bands on a gel to determine the sequence, scientists now analyzed fluorescence and so a program called 'Phred' was developed to make accurate base calls from the fluorescence intensity plots.
But a huge advancement in Phred was the inclusion of a quality score for each base call that it made.
We lovingly refer to these today as Q-scores!
These Q-scores are a log based estimation of the quality of the call.
Phred determined this originally based on an error estimation that took into account the peak height and shape of the fluorescence 'trace' for each base.
Today, these scores are calculated somewhat similarly in that they're still the log of an error estimation, but the factors that go into that estimation can be quite complicated!
The Q-scores we deal with most frequently range from 10-50 and they have the following accuracies:
Q10 - 90%
Q20 - 99%
Q30 - 99.9%
Q40 - 99.99%
Q50 - 99.999%
'So is there a benefit to having a higher Q-score or are they just a marketing scam?'
That's complicated.
They're not a scam since most pipelines Q20 or Q30 trim their base calls to be sure that only bases with a quality of >99% make it into downstream analysis.
'But is there a difference in the usefulness of a Q50 dataset over a Q20 dataset?'
It really depends on the application.
Something we hear about constantly in the whole genome sequencing space is the need for 30x coverage for us to make heterozygous (het) variant calls.
This is important because, if you remember, your genome is composed of two copies of DNA: one from your mom and the other from your dad.
So every position in your genome can be homozygous (same sequence from both parents) or heterozygous (different sequence from each parent).
Interestingly, sampling statistics (math) say that to be >99% certain of a heterozygous base call, you need to look at each position in the genome 30 times.
But even more interestingly, if you look at plots of variant call accuracy vs coverage for Q20, Q30, or Q40 data, they all converge on ~30x coverage for >99% het variant calling!
'So it is a scam!!!'
No, it's just math.
There are applications where higher quality bases are useful, especially in oncology or therapeutics where the variants you care about aren't at frequencies of 50% and 100%, but sub 1% or even lower.
Here, Q40 (99.99%) or Q50 (99.999%) really shine because then the error of the base calls isn't fighting with the frequency of the variants!
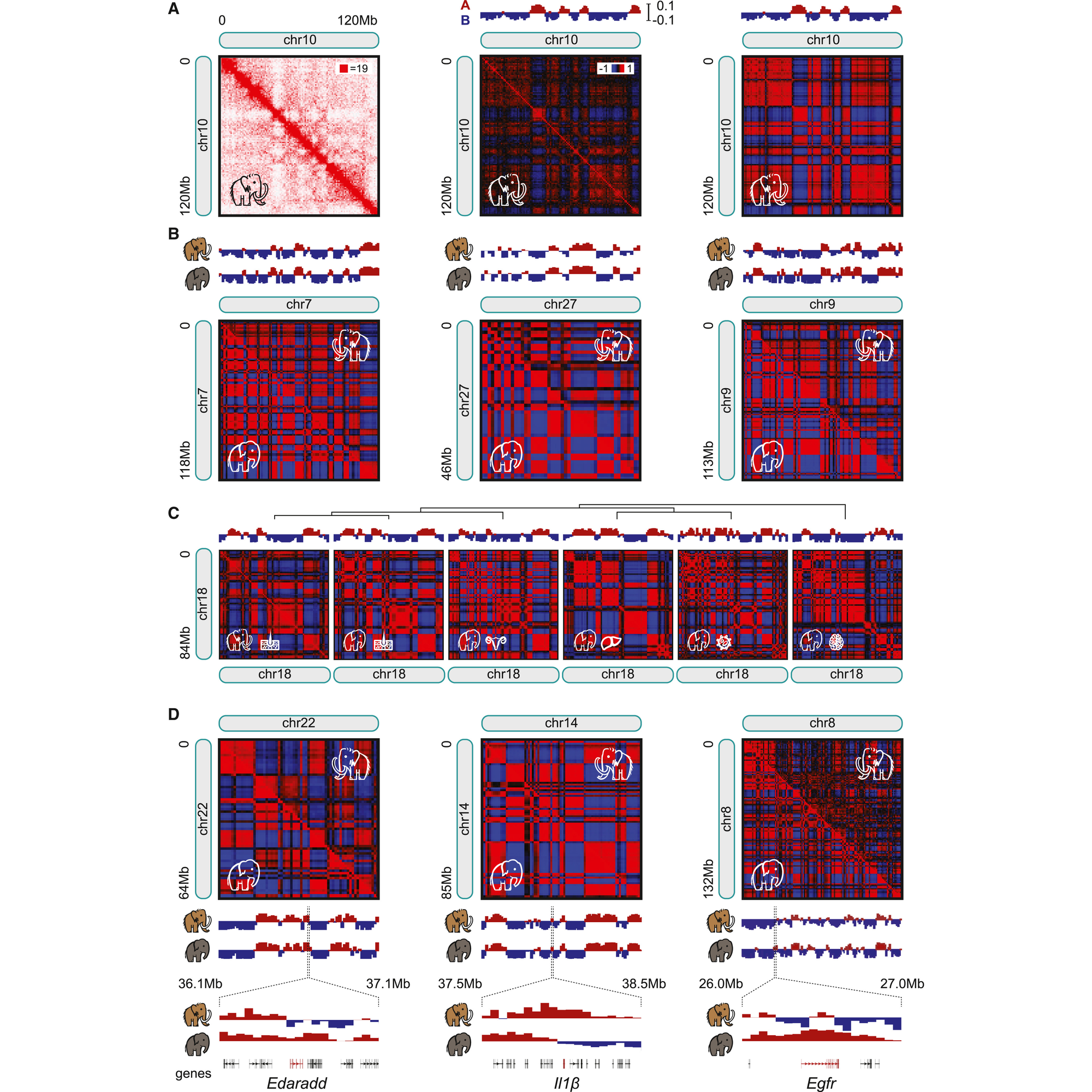