Proteomics is a data deluge. Taming the firehose is still a work in progress.
Once we have all of this amazing proteomic data, what do we do with it???
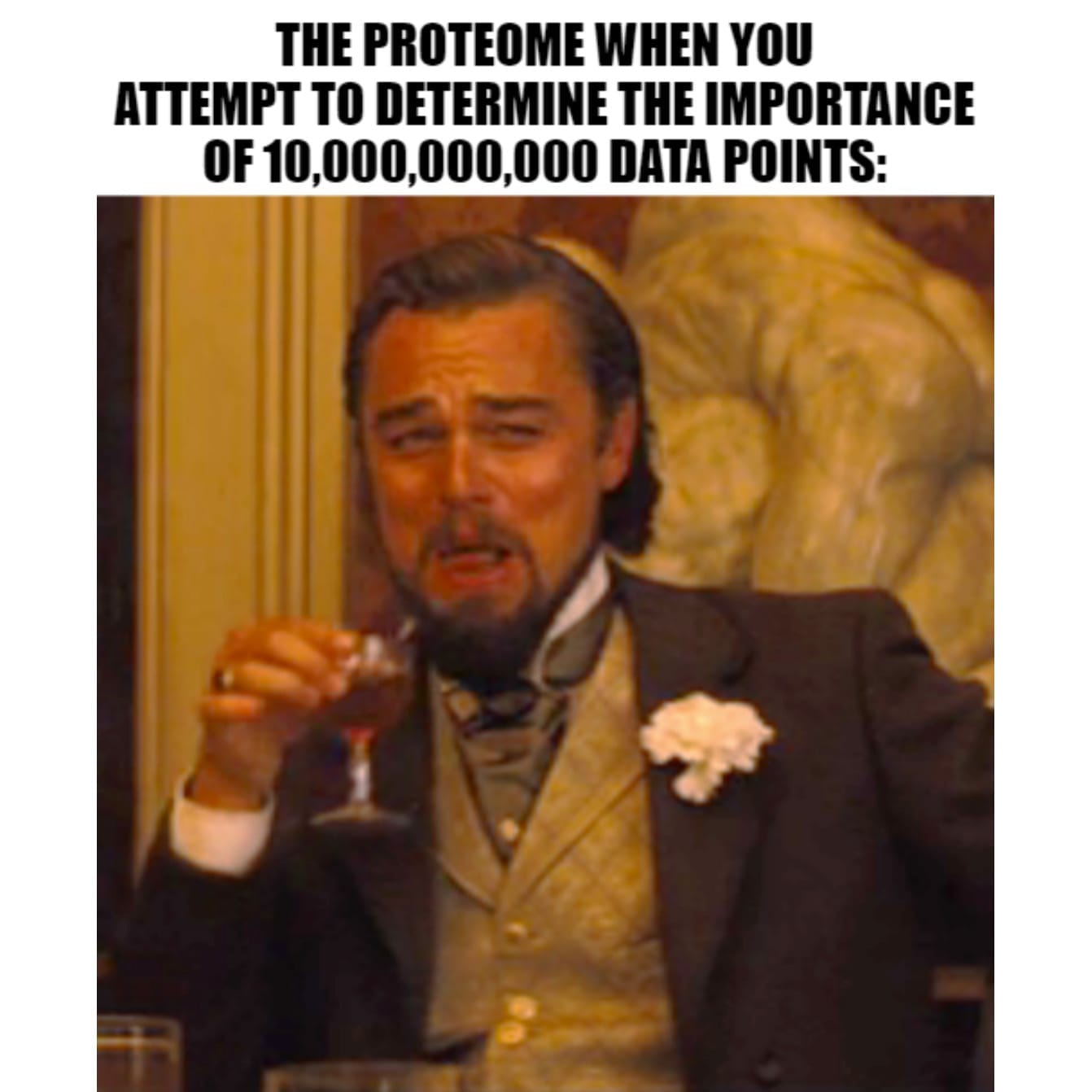
So, once we have all of this amazing proteomic data, what do we do with it???
That's a fantastic question!
But your first question might actually be 'What the heck is proteomics!?'
Proteomics is defined as the study of proteins, their functions, regulation and interactions within an organism.
While the genome holds all of our genetic information, the proteome is the genome in action.
And studying the proteome is quite a bit different than studying the genome because the genome is mostly static.
We have no idea what the impact of a mutation or a variant within the genome will be until we see it manifest as a phenotype (a visible symptom, trait or characteristic).
We can see these things on the molecular level by looking at what proteins are produced!
We can figure this out using a variety of techniques including immuno affinity arrays, mass spectrometry and, in the future, protein sequencers.
But once we've gathered the data, what do we do with it and how can we use it to learn anything?
That answer really depends on the experiment that was performed to generate the data. For clinical applications of proteomics I see 3 types of studies being really important:
Longitudinal studies: it's a big word but it just means looking at how things change over time. For example, these could be used to monitor treatment response in oncology patients or detect flares in Crohn's patients.
Case-control studies: these compare diseased individuals to healthy individuals or, diseased tissues to healthy tissues - looking for differences between the two that could be indicative of health or disease.
Single-cell studies: look at how proteins or their interactions change from cell to cell to get a more granular and nuanced view of tissue function, treatment response, or disease presentation.
Analysis is focused on looking at changes over time, among disease states or across tissues.
But a key first step in doing any of these analyses is normalization!
You want to be sure you're comparing apples to apples and that the differences you see aren't just because of some bias that was introduced.
There are a couple of options here, a popular one is to use a protein that is commonly expressed at a static level.
Once everything is normalized you can start digging in!
Differential protein expression: compare how protein levels change from dataset to dataset. These are usually visualized as heat maps.
Pathway analysis: determine what proteins are present, how they're modified, and how they interact with one another. These are visualized as networks or more recently as circos plots.
But one of the biggest drawbacks of doing proteomics is that we're still creating a knowledge base.
Our techniques for looking at the proteome historically have been very low throughput.
Thankfully, that's changing, and new initiatives are helping to provide proteomic references we can use to better hone our analyses!