Omic.ly Weekly 52
December 2, 2024
Hey There!
Thanks for spending part of your week with Omic.ly!
It's officially the 1 year-a-versary of this newsletter!
Thanks to all of you who have stuck it out for every issue (That's like 2 of you)!
This Week's Headlines
1) RhoFold+: A large language model for RNA structure prediction
2) Direct RNA sequencing might tell us about more than just the bases
3) Oswald Avery, Colin Macleod and Maclyn McCarty showed that DNA was the genetic material in 1944
Here's what you missed in this week's Premium Edition:
HOT TAKE: Just when you thought you might be safe, FDA sticks its nose into MRD testing (again)
Or if you already have a premium sub:
Can LLMs predict RNA structures?
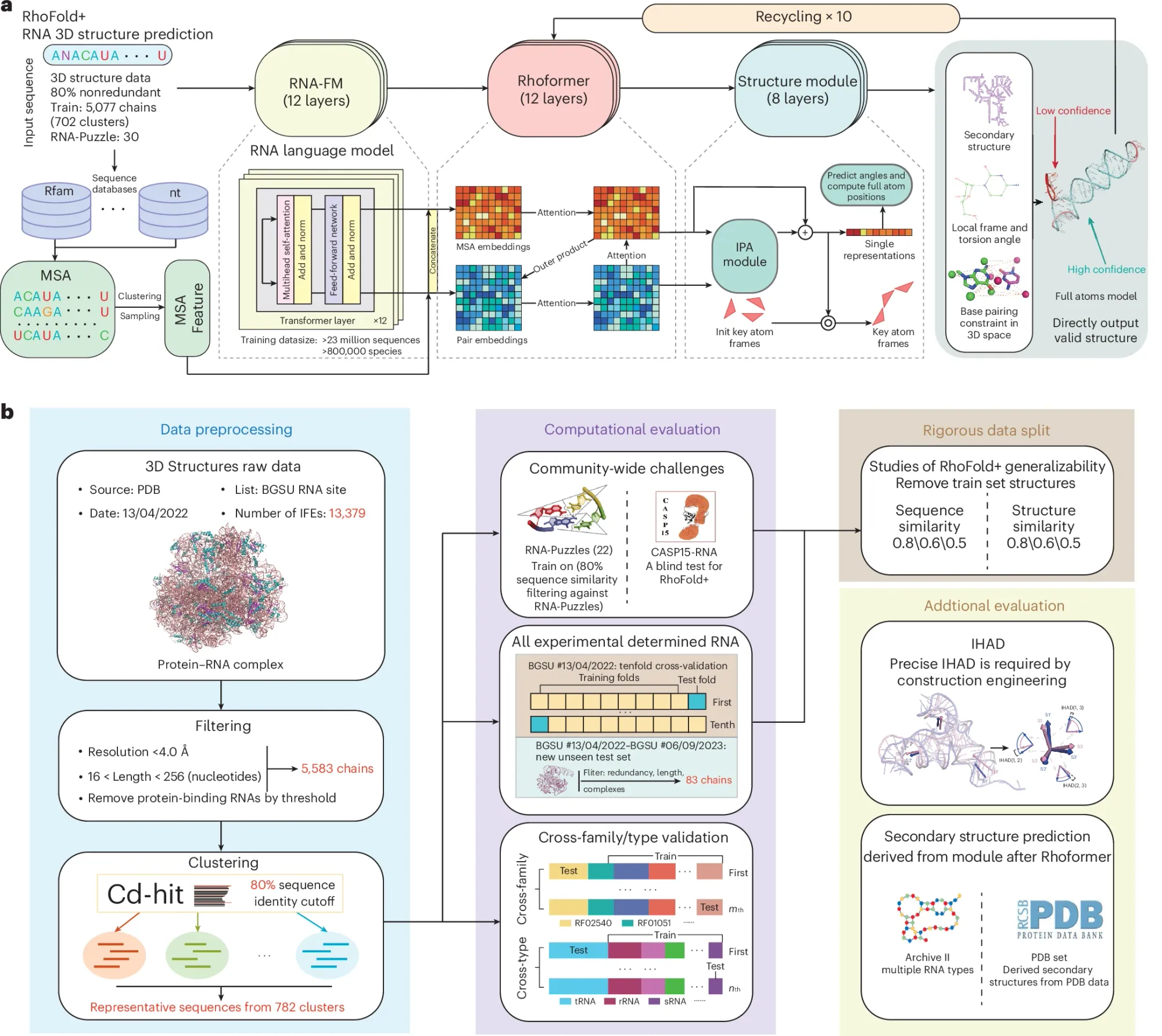
They certainly can try!
One of the biggest disappointments when AlphaFold 3 came out was that it wasn’t very good at predicting the structures of nucleic acids.
Despite it being billed as a structure prediction algorithm for all things biomolecules, the limitations of AlphaFold 3 and other structure prediction models has become increasingly apparent.
And as much as we want all of these things to work like a calculator, where the inputs produce a reliable (and correct) output, AI models aren’t quite there yet.
Although, it’s probably asking a lot for a model to ever be 100% accurate, because that’s the entire essence of a prediction model…sometimes they're wrong and you need to double check what they spit out (for forever).
But that doesn’t mean AI models are useless, or that they won’t get pretty close to perfection, it just means we need to do more iterations, feed them better data, and understand that a model isn’t validated until someone looks to see if the results it produces are correct!
One good way to do this is to benchmark the prediction models against known structures including structures that an algorithm has been trained on.
Because if it can’t get close to the right answer on a training set, there’s no way we’re ever going to be able to believe that what gets spit out for something completely new is worth following up.
This is especially true for things like RNA which can be extremely tricky to predict since nucleic acids can dynamically form many different structures.
But the structures formed by nucleic acids like RNA are important because they can affect how genes are expressed or even how RNA catalyzes reactions in our cells!
So, being able to accurately predict these structures is a big deal.
The authors of this week’s paper attempted to develop a model that could best AlphaFold (and others!) at predicting RNA structures and they named the tool they created RhoFold+.
It’s described in the figure above:
a) It uses a large language model trained on RNA sequence and structure data that were then refined for ten cycles in their transformer network (Rhoformer) and finally that data went through the structural prediction module that assigns the locations and angles of the atoms in the RNA backbone.
b) Is a graphical abstract of the paper and provides an overview of how they selected the data that went into the model and how they validated its outputs.
Their benchmarking study showed that it performed significantly better than other RNA folding algorithms in the aggregate (but was not perfect), was less computationally intensive than its competitors, and its performance on experimentally determined structures could warrant its use on unseen structures.
But the authors were quick to highlight that the dynamic nature of RNAs make them hard to predict, so any work on unseen structures should be experimentally validated.
###
Shen T, et al. 2024. Accurate RNA 3D structure prediction using a language model-based deep learning approach. Nature. DOI: 10.1038/s41592-024-02487-0
Post-transcriptional modifications: Why sequencing RNA directly is the future of transcriptomics
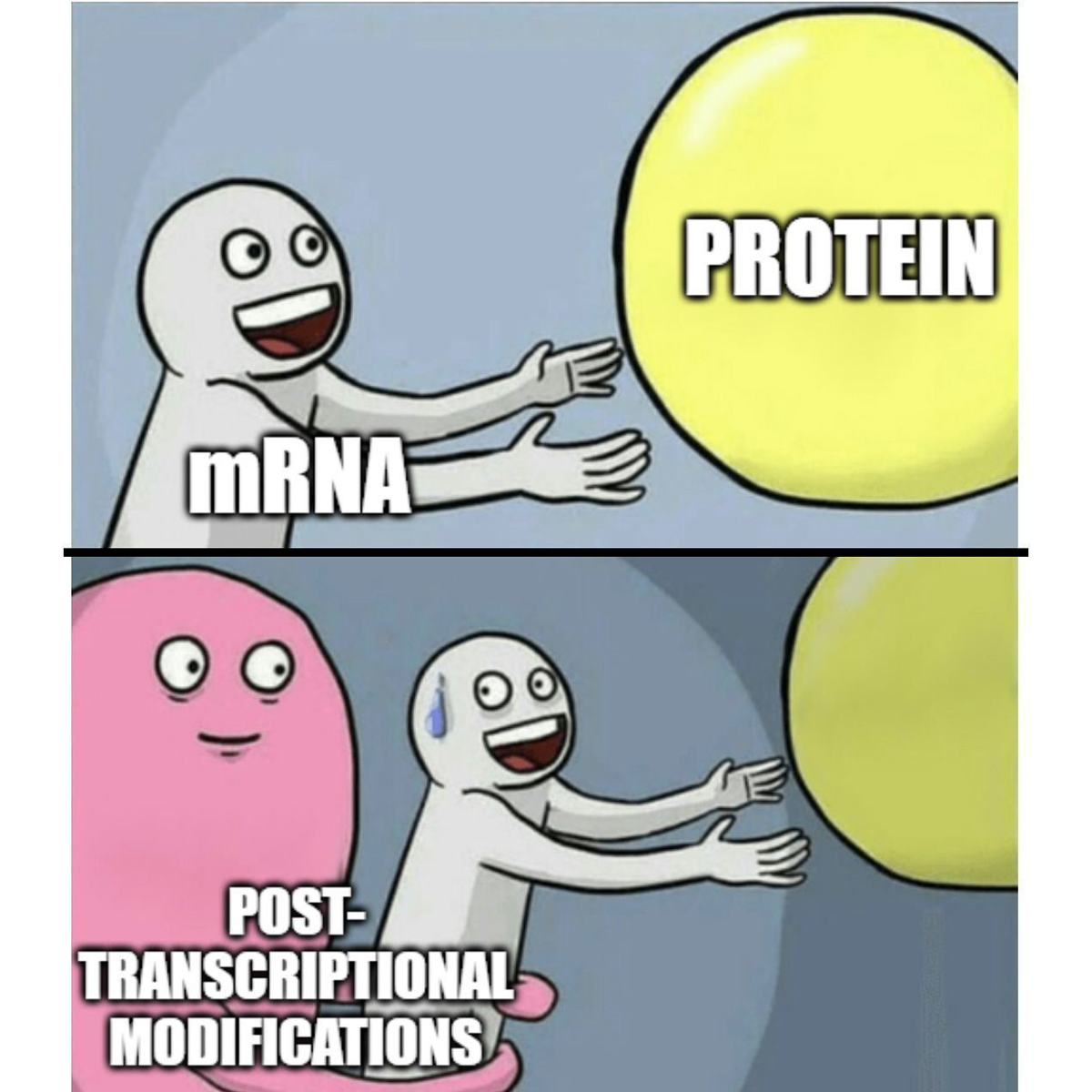
In Francis Crick’s first description of the central dogma in 1957 he stated that genetic information moves in one direction: DNA codes RNA, and RNA codes proteins.
We are now aware of multiple exceptions to this theory but we’ve also started to learn more about how this is controlled at the cellular level.
This is the work of regulatory proteins that bind to nucleic acids and promote or repress the transcription of DNA, or the translation of RNA into proteins.
While the sequences that these proteins recognize are important, we have also learned that modifications to both DNA and RNA play a significant role in these processes.
DNA can be modified through the methylation of cytosine which affects which mRNAs are made (or not made!).
This is one component of Epigenetics!
But RNAs are quite different and we know of over 150 modifications that can be added to them.
So, what are all of these modifications, and what are they doing?
The most common mRNA modification is the addition of a methyl cap made of N7-methylguanosine (m7G).
This cap helps protect the mRNA from degradation but also aids in the binding of proteins involved in its translation to protein.
But residues outside of this cap structure are also frequently modified and include: N6,2′-O-dimethyladenosine (m6Am), N6-methyladenosine (m6A), and pseudouridine (Ψ) with rarer modifications including N1-methyladenosine (m1A), 5-methylcytidine (m5C), 5-hydroxymethylcytidine (hm5C), N4-acetylcytidine (ac4C) and inosine (I).
These all have context dependent effects which are still being unraveled, but we know they are important for nuclear export, regulating translation, or marking specific RNAs for destruction.
We also know that these modifications play a critical role in regulating how cells respond to changing conditions within their environment through sequestering RNAs, ramping up translation, or quickly destroying RNAs to make room for others.
Despite everything we have learned about these modifications, this field of ‘epi-transcriptomics’ is still in its early days.
And that’s because we haven't had a good method to look at all of these things in a high throughput way!
Another contributing factor here is that in traditional transcriptomics, RNA has to be converted to copy DNA (cDNA) to be sequenced and that conversion process doesn’t preserve these modifications.
So, the only way to really survey all of this additional information is to sequence the RNA directly!
Luckily, we have people working on this problem with some early success being reported.
Oxford Nanopore has achieved 95% (~Q13) RNA sequencing accuracy with promising progress in being able to call m6A base modifications!
While there’s still work to do, it's clear that accurately detecting RNA base modifications will be crucial for understanding their role in human health and disease.
One of the most important papers in the history of genetics was basically ignored when it was published in 1944.
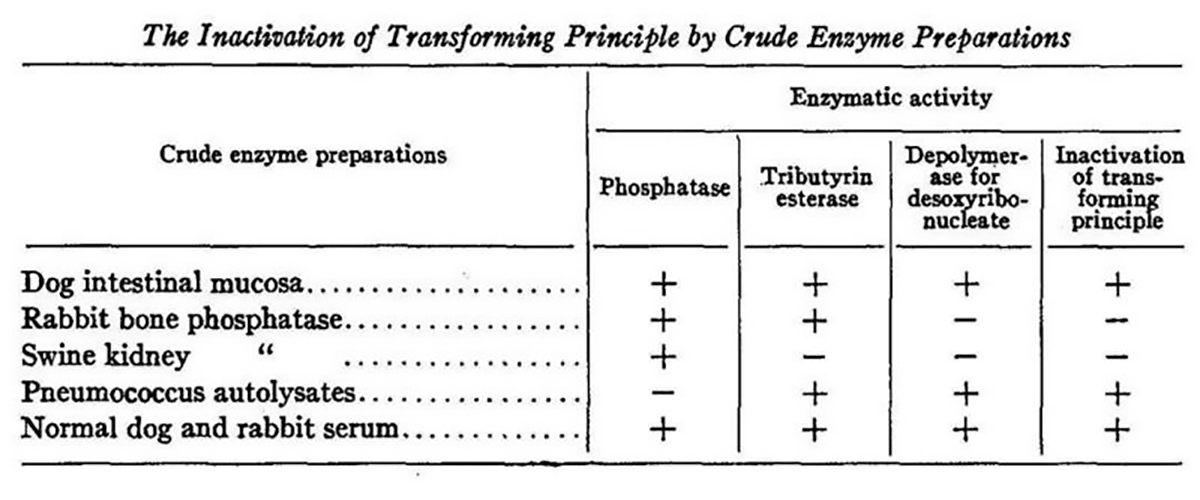
But, before we get to THAT paper, we need to go back one paper to set the stage.
In 1928, Fred Griffith published, ‘The Significance of the Pneumococcal Types,’ where-in he identified the ‘rough’ (doesn’t kill mice) and ‘smooth’ (kills mice) types of pneumococcus (a bacterium).
Through a series of experiments he showed that if you inject mice with rough, they live, and with smooth, they die.
If you heat kill smooth and inject that, they live.
These are not surprising results.
But, if you inject mice with both rough and heat killed smooth, they die.
The killed smooth type is able to ‘transform’ the rough type of pneumococcus into the deadly type.
Griffith’s transformation experiments are considered one of the cornerstones of molecular biology, but up until 1944, basically the entire field of biology thought the ‘transforming factor,’ or genetic material, was protein.
So, suffice it to say that when Oswald Avery, Colin Macleod and Maclyn McCarty published their paper in 1944 showing unequivocally that DNA was the genetic material, it wasn’t very well received.
Basically no one cared.
Mostly because everyone thought the genetic material was protein and that Avery and team screwed up their experiments.
Except, they didn’t.
The figure above shows an experiment where alcohol purified DNA was exposed to five different crude extracts each with different enzyme activities: phosphatase (degrades RNA, RNase), tributyrin esterase (degrades fats and proteins, protease), depolymerase for desoxyribonucleic acid (degrades DNA, DNase).
In this figure you'll see the last column says 'inactivation of transforming principle' and the only extracts that inactivate the transforming principle are the ones that contain DNase activity.
Purified proteases also failed to inhibit the transforming activity.
But at the time, the genetic material question was deemed to be solved, it was protein, and one of the ring-leaders of the ‘protein is the genetic material’ crew was Linus Pauling.
Pauling is one of the only scientists to receive a Nobel Prize in two different disciplines and is the grandfather of modern structural biology.
He was kind of a big deal.
Pauling himself didn’t believe DNA was the genetic material and instead focused his efforts on studying protein structures.
It was the Hershey-Chase experiment in 1952 that finally convinced him that DNA was the real deal but by that time it was too late.
Watson and Crick, two scientists inspired by Pauling’s work, solved the structure of DNA in 1953 (with help from Rosalind Franklin and others).
But you can't feel too bad for Pauling, he didn't need 3 Nobels anyway.
###
Avery, O et al. 1944. Studies on the Chemical Nature of the Substance Inducing Transformation of Pneumococcal Types. J Exp Med. DOI: 10.1084/jem.79.2.137
Were you forwarded this newsletter?
LOVE IT.
If you liked what you read, consider signing up for your own subscription here: