Omic.ly Weekly 50
November 18, 2024
Hey There!
Thanks for spending part of your week with Omic.ly!
This Week's Headlines
1) Nanopores are getting on the rapid NICU/PICU sequencing bandwagon
2) New tech always has risks and limitations, single-cell and spatial transcriptomics are no exception
3) A wormy discovery becomes a knockout laboratory technique
Here's what you missed in this week's Premium Edition:
HOT TAKE: A four legged adventure into the world of cancer vaccines
Or if you already have a premium sub:
Nanopores take on rapid neonatal sequencing
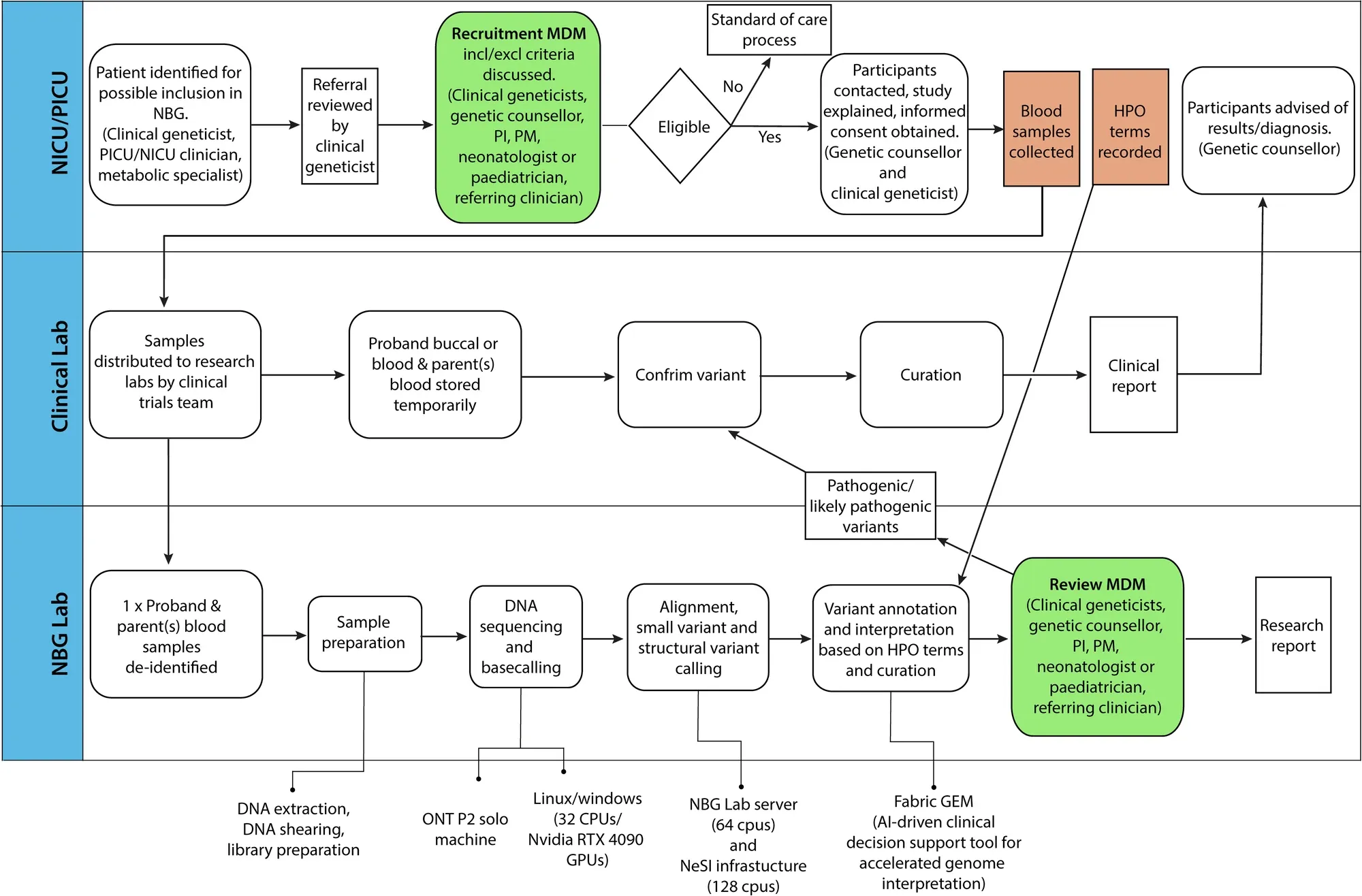
When it comes to diagnosis of rare, early on-set genetic disease, speed to diagnosis can make a huge difference in a child’s quality of life post-diagnosis.
This is why we’ve seen an explosion of stories (and studies) about rapid neonatal sequencing.
Much of this work was (and still is) championed by Dr. Stephen Kingsmore of Rady’s Children Hospital who debuted a 26hr genome in 2015 using a modified Illumina HiSeq 2500 to rapidly diagnose children in the Neonatal Intensive Care Unit (NICU).
But, one of the major limitations of short-read genome sequencing is that it misses things.
This is especially true with respect to mutations in pseudogenes, paralogs, variants that occur in repetitive sequences, and larger structural variants like inversions and translocations.
While short-read bioinformatic technologies have improved over the years, they’re still not as good at picking up all of the variant classes that we care about.
This means that kids who are not diagnosed with short-read whole genome sequencing still have to endure a diagnostic odyssey to get a final diagnosis.
This is usually done using follow up testing with things like microarray, methylation sequencing, or Multiplex ligation-dependent probe amplification (MLPA) to detect structural variants in problematic genes.
One way around needing to do all of this extra work in undiagnosed cases is to use long-read sequencing technologies such as those offered by Pacific Biosciences or Oxford Nanopore Technologies.
These sequencers look at multi-kilobase long fragments, and can also natively detect methylation patterns.
However, up until just a few years ago, these long-read technologies were prohibitively expensive and error prone.
Today, they’ve caught up with short-reads on both cost and quality.
And because they can access longer range information and detect methylation, they can diagnose cases that short-reads would miss!
So, why aren’t we using these superior technologies in the clinic?
That’s mostly because short-reads have about a decade long head start, and adoption of new technology in healthcare is excruciatingly slow.
The way we get around this is to generate clinical evidence to show that long-reads perform as well as or better than short-reads by comparing their performance in the clinic!
We’re just starting to see these studies come out now, and one was published last week.
In this study, the clinicians used Oxford Nanopore sequencing on a P2 solo device to benchmark the performance of the sequencer against 6 well characterized genomes (HG002-7) and showed high levels of precision and recall for single-nucleotide variants (SNVs) (>.99) and small insertions and deletions (Indels) (>.8).
They also showed that the native methylation calls had high concordance with whole genome bisulphite sequencing on the same samples.
Finally, they developed a pipeline (which can be seen in the figure above) to perform rapid sequencing of neonatal patients in their NICU and showed a comparison of the first 10 results obtained using the rapid nanopore based method and more traditional Illumina short-read sequencing.
In the 10 cases presented, the rapid nanopore sequencing identified the same variants as the short-read method and diagnoses were obtained in 6 of the patients sequenced.
While this is a small study and not a full clinical validation of a nanopore based diagnostic pipeline, it’s a promising step in the right direction to help bring long-reads into clinical practice.
###
Nyaga DM, et al. 2024. Benchmarking nanopore sequencing and rapid genomics feasibility: validation at a quaternary hospital in New Zealand. DOI: 10.1038/s41525-024-00445-5
Single-Cell and Spatial Transcriptomics: Risks, Limitations, and Questions to Ask.
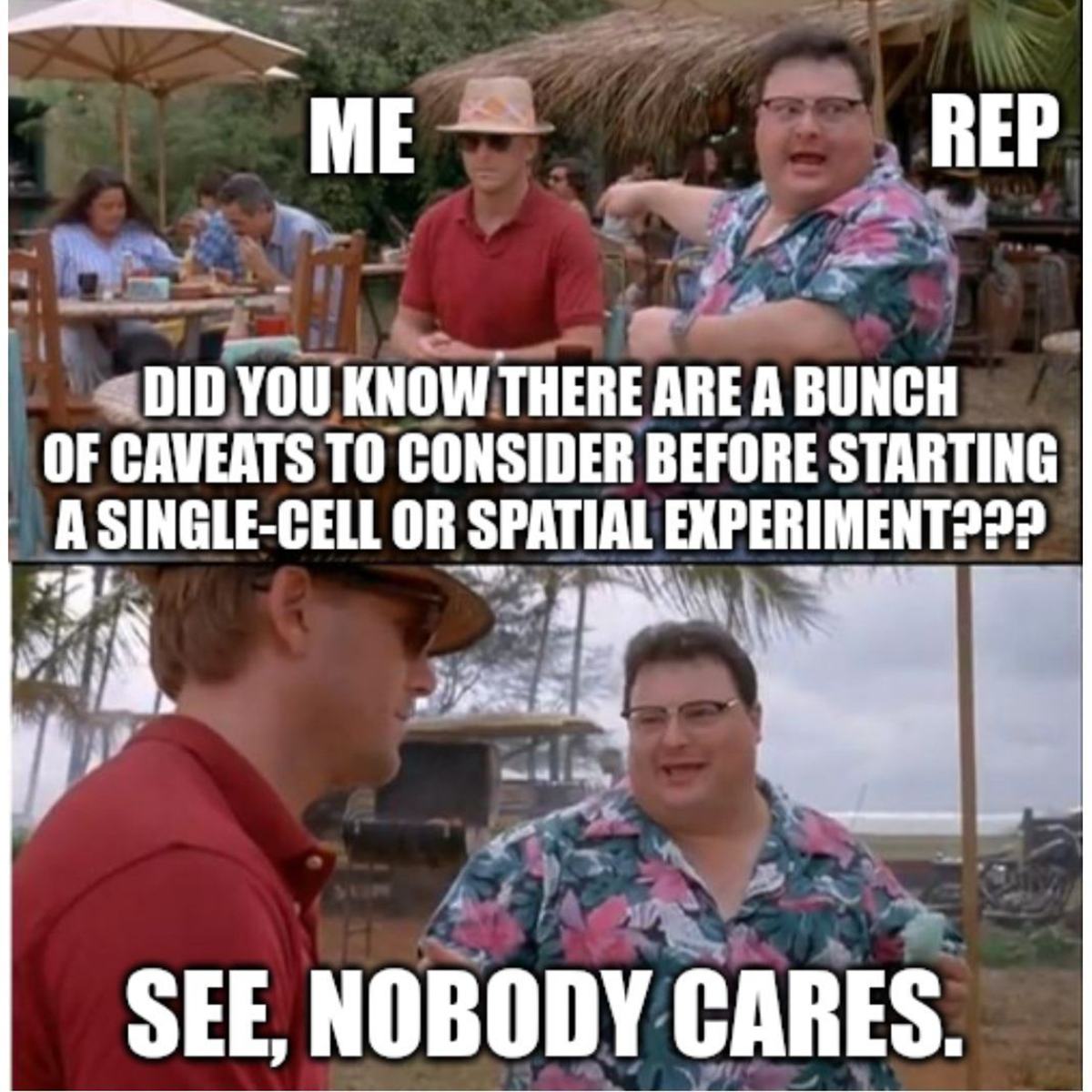
"Brian, why do you always have to be such a wet blanket?"
Ah, the age-old question...
But this wouldn't be science if we didn't also think about all the ways these things might not be telling us the whole story.
Or worse, telling us the wrong story!
Here are a few things to consider before you make a bunch of pretty pictures or tSNE plots:
Abundant Transcripts - Some RNAs are expressed much more than other RNAs. These are usually things like ‘housekeeping’ genes that are involved in supporting major cellular functions, for example, ribosomal RNA. These signals can dominate a dataset and hide information you care about seeing.
Sensitivity/Specificity - Do you pick up all the positive signals, are the negatives actually negative? Do you have enough reads/resolution to know? Controls are your friend here but not all controls are created equally! Is anyone working on synthetic single-cell controls (Are they??)?
Full-length vs 3'-UTRs/Hybridization Probes - Do you care about knowing what isoforms are present in your samples? Choosing the right methodology here is key because alternative splicing of transcripts can change what story you might tell if you only look at probe binding/sequencing from 3'-UTRs!
Recovery - This is single-cell specific, but the quality of your input cells and the technology you use can dramatically affect how many cells you actually get data from. Do your handling processes bias recovery? Do you have enough input to recover a representative population of cells?
Spatial Specific Limitations:
Resolution - Spatial resolutions are all over the place from 10um down to 100nm! That's a wide range and it means that in the lower resolution methods there's a chance you can get signal overlap and miss things that you might want to actually detect. Which leads to the next problem…
Fluorescence Interference/Crowding - Are you detecting the signals you think you're detecting or is there interference or crowding because two things you care about occupy the same space?
Transcript Accessibility - Are the RNAs you're trying to detect accessible for probe or sequencing primer binding? What if they're covered in proteins or twisted up? Does that mean you don't detect them!? The inside of a fixed cell is a messy place…
Quantifying Spatial Results - Some methods naturally capture results in a matrix or a grid which makes it slightly easier to know that your results are quantified properly but what about methods that claim cellular or subcellular localization? Can they actually accurately determine cell boundaries? Sometimes that answer is yes.
"So, Are you saying everything we're doing is worthless?"
Nope, plus this is a rapidly evolving field that's improving every day!
But you should think really hard about how you're going to perform your experiments and be sure to validate your conclusions with secondary assays.
One of the best ways to figure out what a gene does is to get rid of it and see what happens.
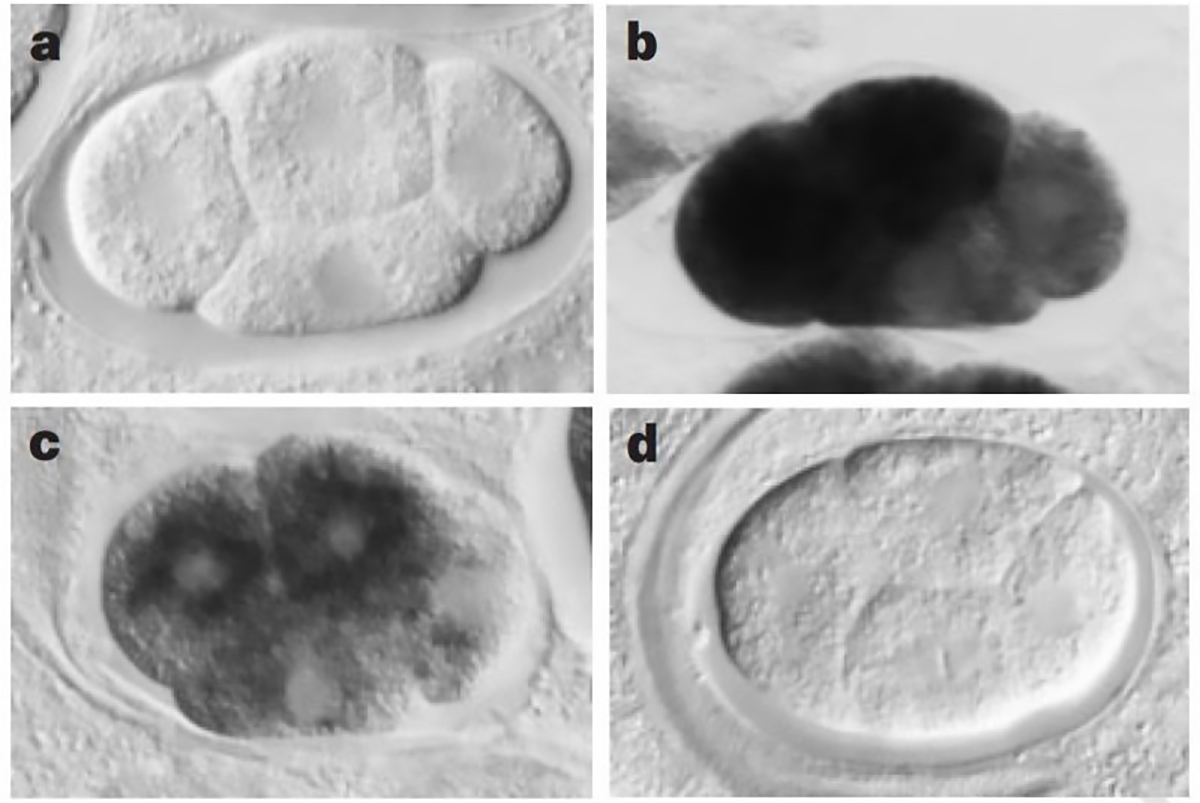
Prior to 1998, this was time consuming and tedious.
That all changed with the discovery of RNA interference.
Gene expression is the process by which the genetic code is converted into something that performs a function within the cell.
Most of the time, this means the conversion of DNA into messenger RNA and then messenger RNA (mRNA) into protein.
It had been known for some time that gene expression could be modified through the introduction of 'anti-sense' or complementary RNA. It was thought that this RNA would bind to the mRNA and prevent it from being read to create protein.
While useful in some biological studies, the effect of anti-sense RNA treatment was modest and the mechanism of how it worked was poorly understood.
So, in 1998, Andy Fire and Craig Mello embarked on a journey to see if they could make this gene expression modification tool more efficient, hypothesizing that the structure of the anti-sense RNA might play a key role.
What they discovered surprised them.
Being good scientists, they introduced anti-sense RNA, sense RNA and both anti-sense and sense together as a double-stranded RNA (dsRNA) complex to determine what effect these combinations would have on gene expression.
The sense and anti-sense treatments had modest effects on their study subject, the nematode worm, C. elegans, but the inclusion of the dsRNA combination had a striking phenotypic effect, mimicking what would have been expected if the genes they were targeting were totally knocked out.
The figure above shows a series of images of a C. elegans embryo. Panel A is the negative control; Panel B shows the staining of an abundant mRNA, mex-3; Panel C shows what happens to mex-3 RNA in the presence of anti-sense RNA; and D shows the complete obliteration of mex-3 RNA after the injection of dsRNA.
Further work after this 1998 paper resulted in the discovery that RNA interference is actually a biological process that cells use to regulate the expression of genes, allowing scientists to identify and name a bunch of new proteins like Drosha, Dicer, Argonaut and the RNA-Induced Silencing Complex (RISC).
We have since co-opted this process for our own uses as a scientific tool and also as a therapeutic for treating human diseases.
Unsurprisingly, given its utility and continued impact, Andy Fire and Craig Mello received the Nobel Prize in Physiology or Medicine in 2006 for their discovery of RNA interference.
###
Fire A et al. 1998. Potent and specific genetic interference by double-stranded RNA in Caenorhabditis elegans. Nature. DOI:10.1038/35888
Were you forwarded this newsletter?
LOVE IT.
If you liked what you read, consider signing up for your own subscription here: