Omic.ly Weekly 49
November 11, 2024
Hey There!
Thanks for spending part of your week with Omic.ly!
This Week's Headlines
1) AI takes on virus evolution
2) Applications of single-cell and spatial transcriptomics
3) Fred Sanger didn't get his first Nobel for DNA sequencing
Here's what you missed in this week's Premium Edition:
HOT TAKE: A round-up of everything that happened this year at ASHG
Or if you already have a premium sub:
AI to the rescue: using protein structural similarities to track virus evolution.
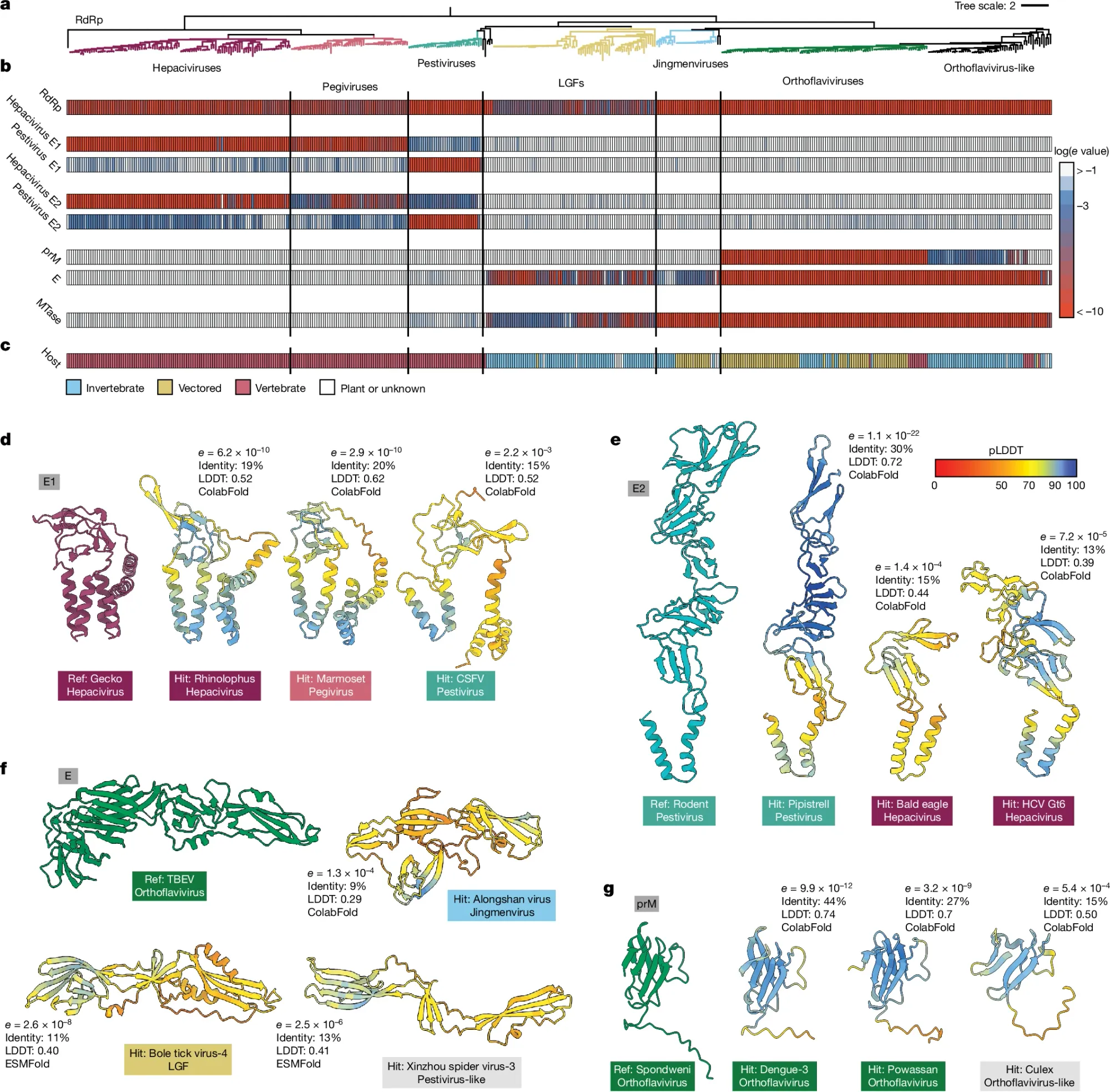
How do we know that organisms are related to each other?
Prior to the invention of DNA sequencing, a lot of that guess work was done by … guessing.
Basically, scientists in olden times looked at two organisms, saw what physical features matched between them, and then decided how related they were.
This, of course, is a gross oversimplification and actual measurements of the sizes of these structures and their appearance throughout the fossil record were used to place organisms into phylogenetic trees.
Charles Darwin famously created such associations using the characteristics of finch beak size on the Galapagos Islands to track the evolution of these birds from island to island.
But now that we’re in the age of genetics, we also include molecular genetic information during the process of creating these trees.
So, both physical characteristics and genetic characteristics are used to create the evolutionary trees that explain how something like a human shares a distant common ancestor with the great apes!
But how do we figure out the evolutionary history of things where their physical characteristics aren’t obvious, like in viruses?
Up until now it’s been based primarily on sequence similarity (and how they look under a microscope), but we have found these sorts of analyses to be challenging because viruses evolve (genetically) extremely quickly.
They have very short life cycles and are usually under extreme selection pressure ie immune systems don’t like viruses and try to kill them.
This means that viruses with little genetic tweaks that allow them to evade the immune system of their host can survive to live another day!
But this also means that their genomes can be a totally jumbled mess that makes it hard for us to figure out how they’re all related!
Wouldn’t it be cool if viruses had physical characteristics that we could use in concert with genetic data to help us with that detective work?
Well, viruses, like all other organisms, are basically just bags of nucleic acid and proteins.
As we’ve established, we can sequence DNA and RNA, so how can we use the proteins?
We could generate crystal structures of them from all of the viruses, but that would be an ungodly tedious task.
What if we could use protein folding AI to do that dirty work for us instead?
That’s the basic premise of this week’s paper where the authors went through an exhaustive characterization of the flaviviruses (includes all-stars like HepC, Zika, and Dengue) and found some exciting new relationships that would have been impossible to identify only using genetic sequences.
They did this by feeding sequences and protein structures into AlphaFold2/ColabFold and ESMFold to generate protein structures and then used FoldSeek to look for structural similarities among the proteins contained within the entire Flaviviridae proteome.
This can be seen in the figure above where in a) the researchers looked across all of the flaviviruses, b) chose a set of 8 proteins to compare for homology (Red - high homology, blue - low homology, white - no protein found), c) shows what host each virus infects, and d-g) show representative protein structure comparisons.
They then used these protein homologies to generate a new phylogenetic tree for the flaviviruses.
They also found that some flaviviruses stole a protein, Rnase T2, from bacteria!
While these results are scientifically interesting, they also have implications in the clinic.
Because, now we can use the knowledge that genetically distinct viruses might actually share similar protein structures and therefore might be susceptible to similar therapies!
But, this paper also highlights the importance of remembering that DNA and amino acids are like LEGOS, you can combine them in lots of different ways to make functionally similar things.
And that applies to all organisms, not just viruses!
###
Mifsud JCO, et al. 2024. Mapping glycoprotein structure reveals Flaviviridae evolutionary history. Nature. DOI: 10.1038/s41586-024-07899-8
Single cell and spatial transcriptomics: Let's explore some applications!
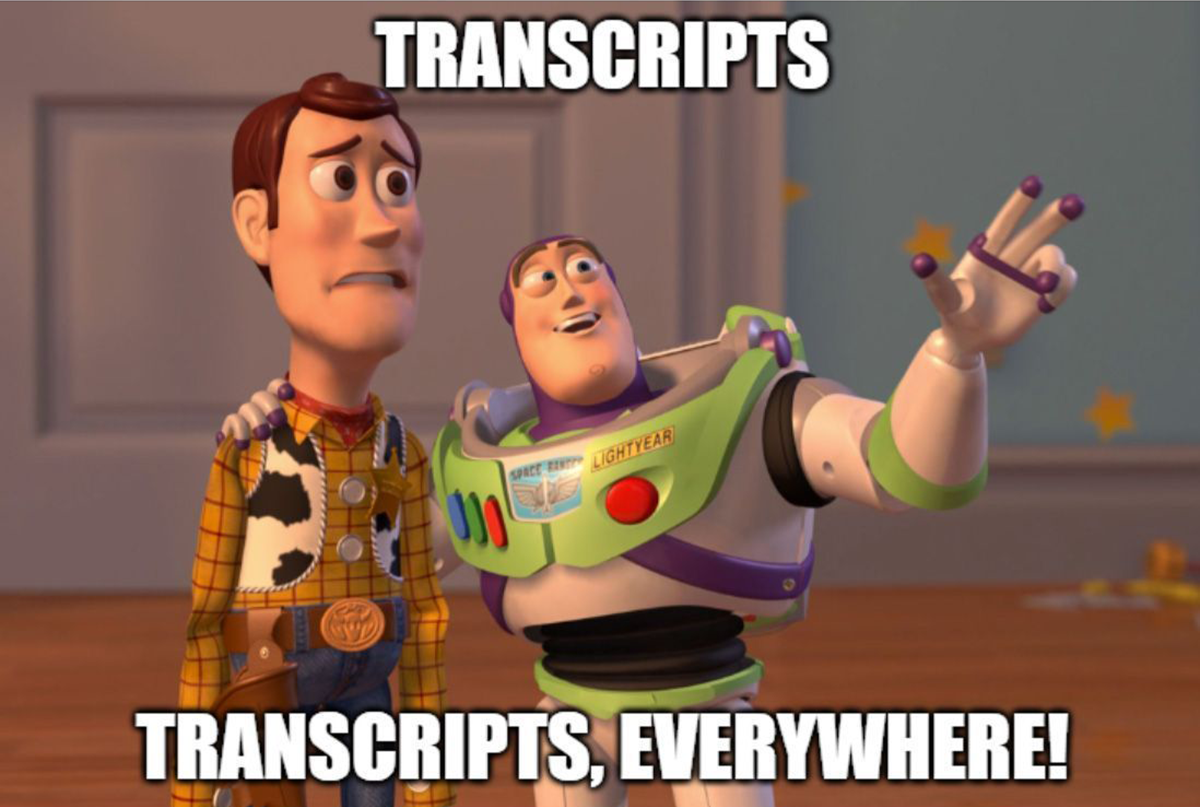
Transcriptomics is the study of the all of the RNA sequences that are present within a cell.
The transcriptome is a product of our genome and the differences in RNA expression that we see across cell types is responsible for all of the functions performed by our tissues which themselves are made up of trillions of cells all with slightly different transcriptomes.
So for us to really, actually, understand anything about what our genome does, we need to look at the functional output of the genome.
One of those outputs is the transcriptome!
Spatial and single cell transcriptomics takes this idea one step further by singling out the expression of RNA in single cells or within specific tissue environments.
These techniques have use cases in a wide variety of scientific disciplines.
Developmental Biology: multicellular organisms develop from, at a minimum, a single cell, so how does a single cell turn into an entire organism? It's almost always a highly complex regulatory dance between the genome, the RNA and proteins it encodes, and the feedback loops that are established to regulate what is expressed by each cellular genome in a specific tissue micro environment. The only way we can understand this better is with cellular and spatial read outs of the functional biology.
Oncology (cancer): both solid tumors and leukemias (blood cancers) are perfect targets for single cell and spatial transcriptomics because the best way to attack a cancer is by better understanding its biology and its weak points. If we know what is expressed or not expressed, that can give us insight into how best to develop drugs to stop its spread.
Immunology: blood cell lineage differentiation is complex and the best way to understand how we go from stem cells to the 12-ish types of blood cells and all of their associated leukemias and autoimmune/inflammatory diseases is by looking at what genes are expressed within those individual cells.
Pathology: this is the realm of the dark art of tissue biopsies and microscope slide staining techniques. While we can learn a lot about disease just by looking at cellular morphology, if we add in transcriptomics and proteomics, we can start to understand how diseases go awry at the genetic level, opening up possibilities for developing therapeutics for specific cell types within a diseased tissue.
The biggest challenge though is the same challenge in everything Omics: does the cost justify the clinical benefit?
Certainly in some cases that answer will be yes, but the cost of processing a pathology slide is easily 1/100th the cost of doing single cell and/or spatial transcriptomics!
So, unfortunately, there's a good amount of clinical evidence generation ahead of us before we see broad adoption of these techniques in the clinic.
Fred Sanger received a Nobel Prize for his work with Insulin. As the father of DNA sequencing, this surely was for insulin's nucleic acid sequence? It wasn't.
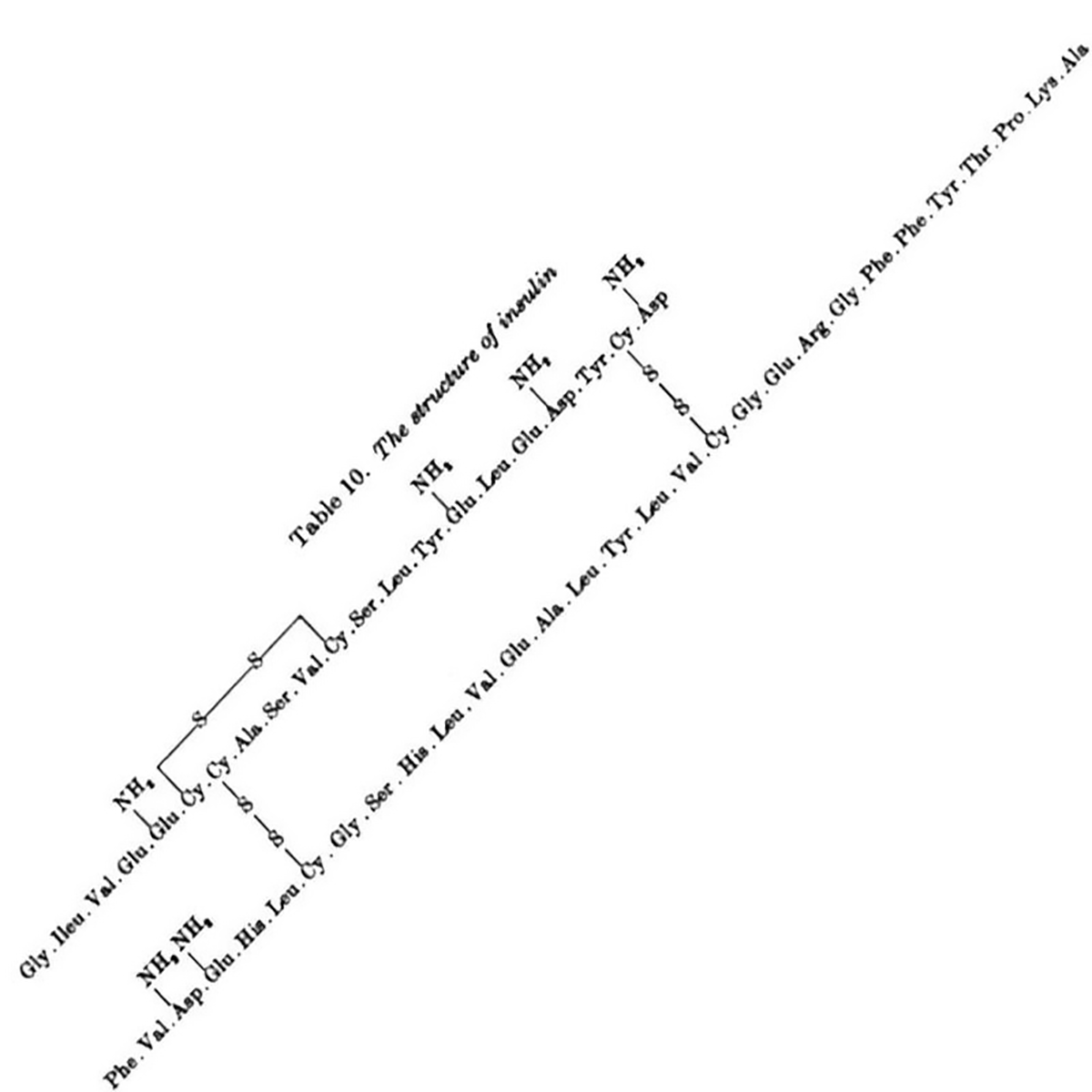
DNA sequencing was his second Nobel.
His first was for the amino acid sequence of insulin.
To say Sanger was an accomplished scientist is an understatement and, unfortunately, his early work sequencing insulin doesn't get nearly the attention that it deserves.
But the experiments leading up to this Nobel worthy achievement began in 1943.
As a new member in Charles Chibnall's group at Cambridge, it was suggested that Sanger focus on exploring the amino acid composition of insulin.
At the time, insulin was basically the only highly purified protein available due to its use in treating diabetes.
It was pure luck that insulin was a small protein, but, as is usually true, small packages can be deceiving, and it took 12 years for him to determine its complete sequence.
The majority of this work was done by tagging proteins on the N-terminus using fluorodinitrobenzene (FDNB) followed by acid hydrolysis and/or trypsin digestion and then separation in two dimensions - first by electrophoresis and then by paper chromatography.
Or, more simply, FDNB turns proteins yellow. Sanger then chopped them into smaller fragments, and the separation technique allowed him to count which amino acids appeared in each fragment and to deduce their order.
The final protein sequence was stitched together by lining up all the overlapping sequence fragments.
Sanger discovered Insulin has an A chain and a B chain. He sequenced the B chain first, and published that 30 amino acid sequence in 1951.
The 21 amino acid sequence of the A chain wasn't completed until 1952, mostly because it has an intramolecular disulphide bridge that made sequencing it difficult.
The figure above is the culmination of years of hard work that had to be completely redone using slightly different methods that played nicer with disulphide bridges, but this allowed for the identification of their location within the sequence.
The figure below shows the amino acid sequence of both A and B chains of insulin along with the 3 disulphide bridges (S-S) - one intramolecular within A and two others that connect chain A to chain B.
Importantly, this work settled a debate about the structural nature of proteins which, among some scientific circles, was believed to be somewhat fluid.
Sanger showed that insulin had a specific amino acid sequence and, by extension, this was likely true for all proteins.
This seemingly minor detail is what set the stage for Crick's 1958 hypothesis for how DNA codes for proteins.
Surprisingly, the 'Sanger' DNA sequencing method that we all know and (mostly) love wasn't actually developed until 1977!
###
Ryle AP et al. 1955. The disulphide bonds of insulin. Biochem. J. DOI: 10.1042/bj0600541
Were you forwarded this newsletter?
LOVE IT.
If you liked what you read, consider signing up for your own subscription here: