Omic.ly Weekly 45
October 14, 2024
Hey There!
Thanks for spending part of your week with Omic.ly!
And once again, I apologize for the delay. AT&T was doing major work on their fiber lines this weekend in Asheville and I didn't get internet back until last night.
No internet makes publishing an internet newsletter somewhat challenging!
Thanks Helene!
This Week's Headlines
1) Bacteria are better at math than I am.
2) Transcriptomes are sometimes more useful than 'whole genomes'
3) Linus Pauling and his DNA triple helix
Here's what you missed in this week's Premium Edition:
HOT TAKE: After 13 years, Illumina's 'little sequencer that could' has been replaced by a bigger, faster sibling
Or if you already have a premium sub:
Large Language Models have gotten all the AI buzz lately, but did you know that bacterial neural networks can solve computational problems now?
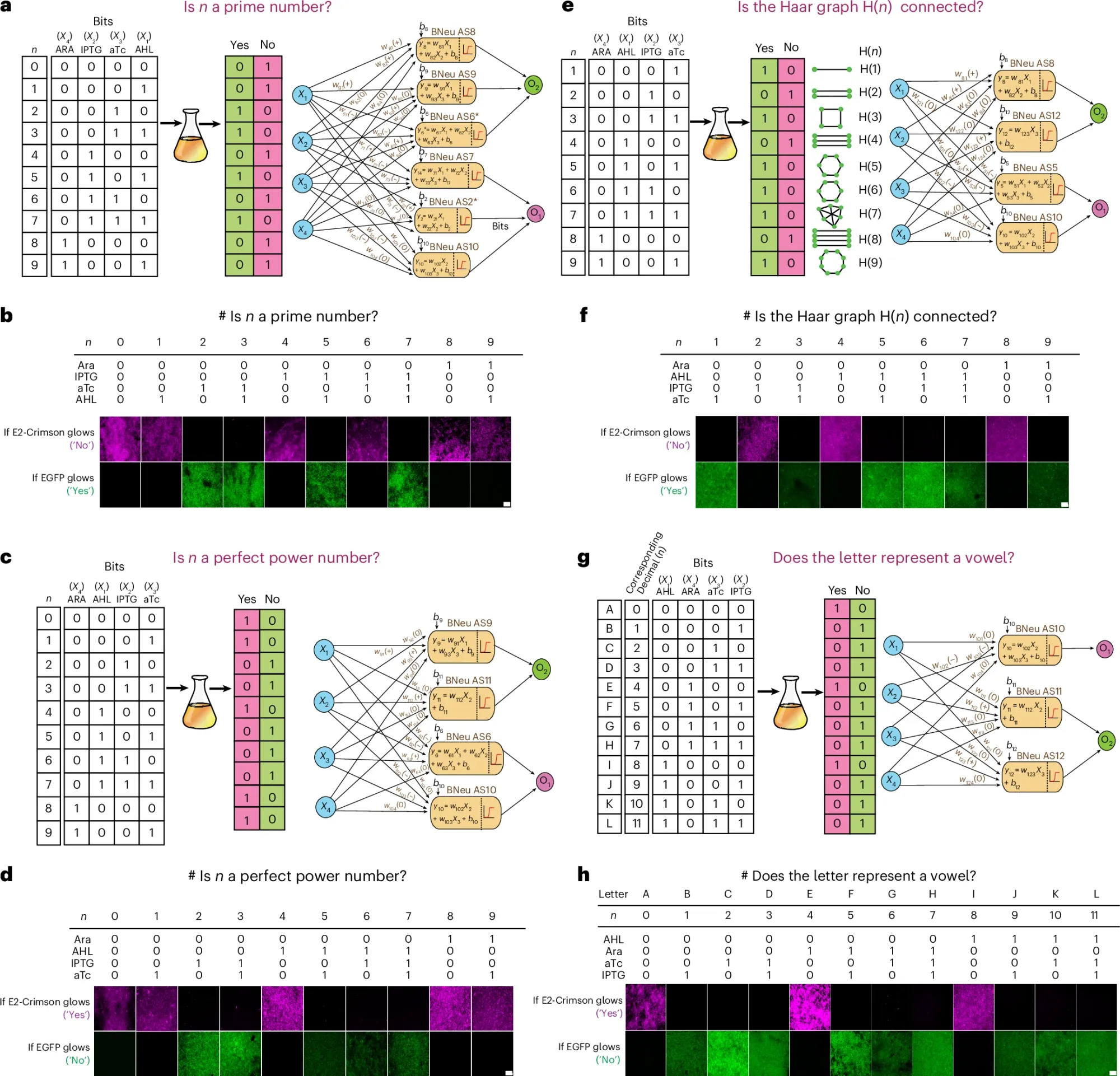
Biological computing has its roots in the work of Leonard Adleman, an American computer scientist (he also helped develop the cryptographic algorithm RSA), who used DNA as a computational system.
In the early 90’s he demonstrated that DNA could solve the ‘traveling salesman problem,’ which is: given x number of cities/customers, what’s the shortest path a salesman has to travel to reach them all?
While this is a relatively simple problem for a human to solve, it’s a classic problem in computational complexity theory and Adleman’s work with DNA showed that biological systems could be programmed to do our digital bidding!
But you might be asking yourself, ‘why would we want biological computers?’
Because, biological molecules are really small!
And since they’re in cells or bacteria, they’re basically self-maintaining.
So instead of running giant, power-hungry, data-centers, what if we could just have bacteria be our computers?
We’re filled with trillions of them, and we provide them with all of the food and nourishment they would need!
Obviously, that symbiotic vision for the future is a ways off, but you can see how in certain scenarios, biological computers could be highly beneficial, particularly as they relate to human health, or as biological sensors for disease early detection and monitoring.
Since they’re so small and self-replicating, bacterial cultures are an attractive place to start trying to figure out how we can create digital biological systems.
The researchers behind this paper used bacteria to create artificial neural networks.
If you’re not familiar with artificial neural networks, they’re computational systems with interconnected nodes that loosely replicate how the neurons in our brain communicate (they receive inputs, transmit signals through layers of the network, and then produce outputs).
These networks can be trained on data and the ‘weights’ of each signal adjusted until they’re performing as expected.
To do this in bacteria, the researchers engineered them to express specific fluorescent signals after exposure to an input (here they used N-acyl homoserine lactone (AHL), isopropyl β-d-1-thiogalactopyranoside (IPTG) and anhydrotetracycline (aTc)).
They then used individual bacteria combined in a co-culture as the nodes, and the ‘weights’ of each signal were adjusted by changing parameters of gene expression regulatory networks ie making promoters more or less efficient, adding more copies of the gene, altering ribosome translation efficiency, etc.
To be honest, it looks like it was a ridiculous amount of work…
BUT, what they were able to achieve was really cool!
In the figure above you can see they were able to ask these cultures mathematical questions and get yes or no answers by looking at how they fluoresced under a microscope!
These are some pretty smart bacteria, because I can only answer two sets of those questions…
And, some of you might notice that these were sent as 4 bit binary signals.
This means that this system isn’t limited to just Yes/No answers!
If you have more than 2 colors for the readout, you can also ask these cultures to solve numerical problems.
And the researchers did just that, showing that the trained bacteria can also do complex math that I DEFINITELY can’t do.
Like, how many slices of pizza are made if you cut it with 7 lines?
Too many, I like big slices…
But, all jokes aside, this is a pretty exciting paper.
Bacterial computers could solve some pressing future computing problems, like, what if a flask of trillions of bacteria could someday outperform our most powerful AI chips?
It might be time to cash in your NVIDIA shares and invest in Luria-Bertani (LB) Broth.
###
Bonnerjee D, et al. 2024. Multicellular artificial neural network-type architectures demonstrate computational problem solving. Nat Chem Bio. DOI: 10.1038/s41589-024-01711-4
Transcriptomes: underappreciated, underutilized, and sometimes more useful than WGS.
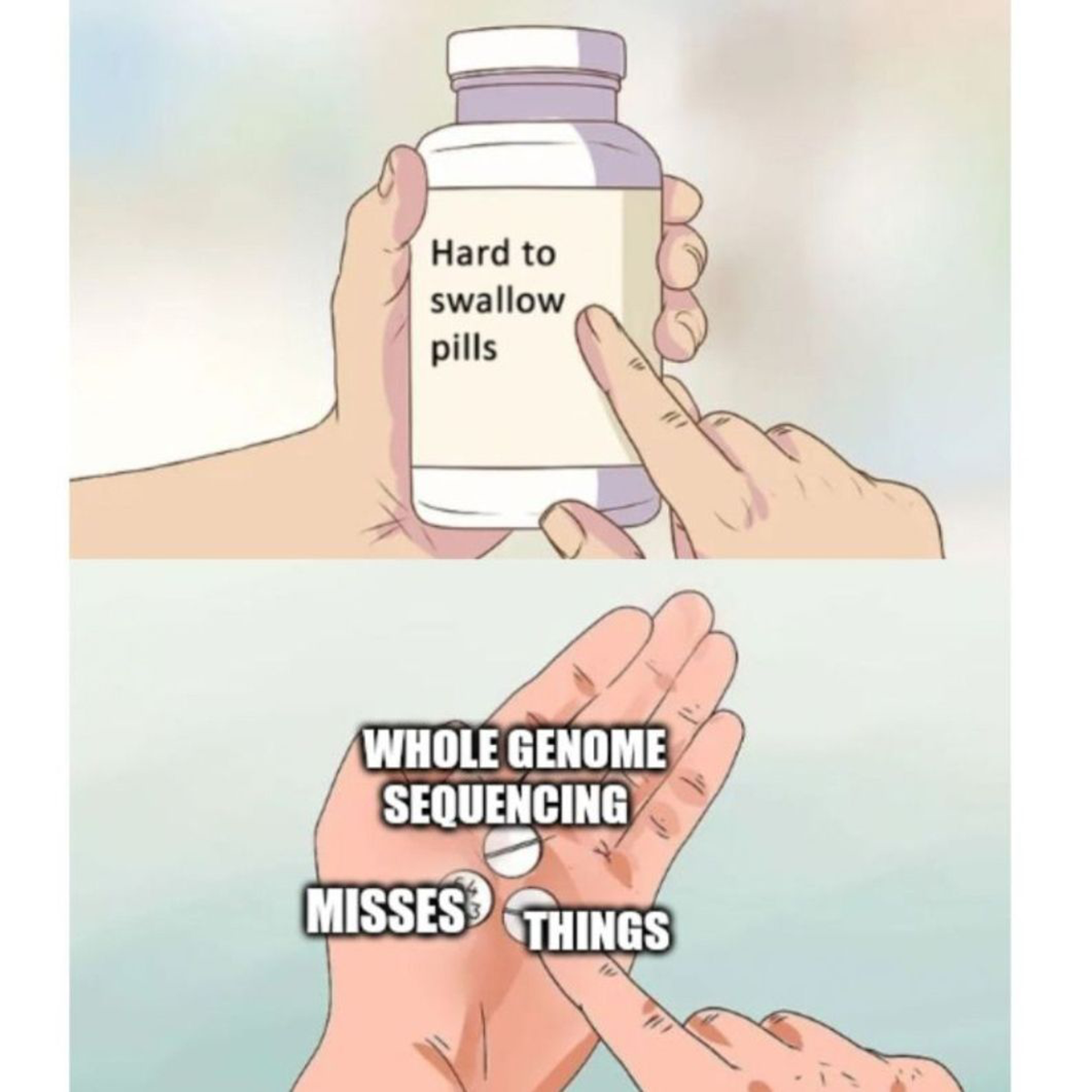
The transcriptome is usually considered a lesser -ome and it’s often overlooked when we talk about rare disease diagnosis, but it shouldn’t be.
Transcriptomics is the study of the all of the RNA sequences that are present within a cell.
The transcriptome is a product of our genome and differences in RNA expression can regulate what proteins are ultimately made.
This determines the functions performed by our tissues which themselves are made up of trillions of cells, all with slightly different transcriptomes.
So for us to really, actually, understand anything about what our genome is doing at any given time, we need to look at the functional output of the genome.
This is especially important in the context of whole genome sequencing because it will find about 5 million variants, the vast majority of those having unknown effects.
And in some of the tougher to solve cases, where a deep intronic or non-coding variant results in disease, we can use transcriptome data to make sense of everything.
Transcriptomes give us 3 important read-outs:
A quantitative measure of gene expression - How much of each RNA is present? Anything missing? More than we expect?
The sequence of expressed transcripts - This is a bit like a double-check of genome sequencing. Do we find differences between what was in the DNA sequence and what is expressed in the RNA? Fusions?
Which isoforms are present - So the genome codes for like 22,000 genes but each of those genes can be alternatively spliced to create more than one version of a protein. 93% of genes undergo this process, so you can see how this can get complicated quickly. And if mutations affect splicing, we’ll see that in what bits of the genome actually make it into the RNA!
The biggest drawback of transcriptomes is that sometimes tissue specific collections are required, especially for muscular disorders, since the cells in our body don't all express the same RNAs.
The kaleidoscopic image below is the triple helix Linus Pauling proposed as the structure of DNA in February 1953. Here's why he got it so wrong:
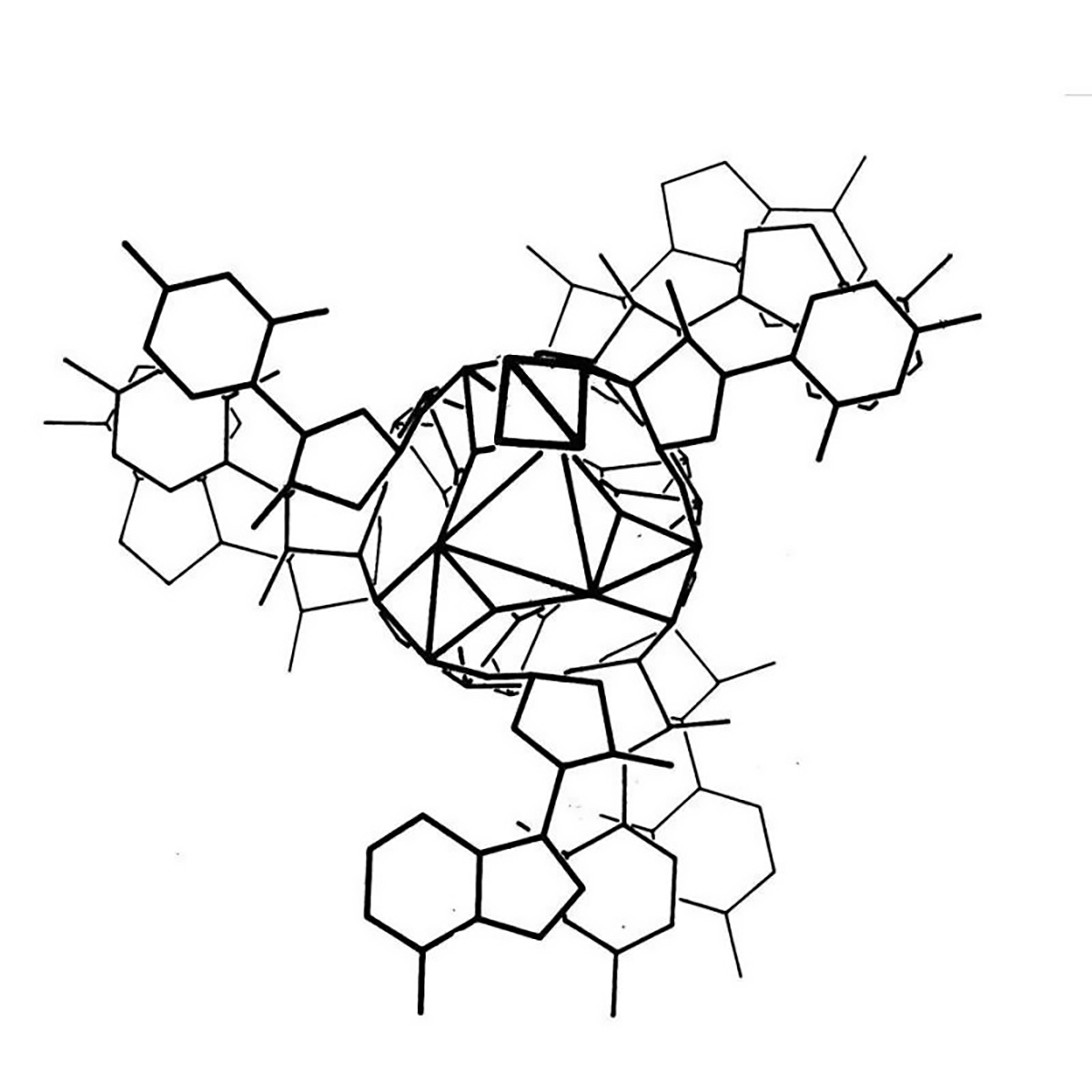
Linus Pauling is remembered as one of the greatest American scientists of all time. He was awarded two undivided Nobel Prizes, and is basically the father of modern structural biology.
He's most famous for solving the 3D structure of the α-helix and the β-sheet, the two most common protein structures.
To do this, Pauling employed a technique called x-ray crystallography which basically bombards a crystal with x-rays. How those x-rays bounce off of a crystal tells you something about the shape of the item that was crystallized and the resulting 'diffraction patterns' can be used to determine key structural features of the crystallized molecules.
In his work, Pauling took this a step further and was one of the first scientists to use that information to model molecular structures with balls and sticks.
Fresh off of his foundational work with proteins, Pauling turned his attention to DNA. While Pauling famously thought that DNA was NOT the genetic material, there's a rumor that he heard that teams in the UK were close to solving DNA's structure and the ever competitive Pauling wanted to see if he could beat them.
However, Pauling didn't generate his own data and relied heavily on data and calculations derived by others in the field, namely those created by William Astbury and Florence Bell in 1938.
Unfortunately, Pauling was unaware of the most recent advancements in the diffraction of DNA by Franklin and Gosling.
But he did have access to electron micrographs of DNA and the 15 year old Astbury/Bell data which indicated the structure was helical.
Based on incorrect density calculations, he also proposed that the structure had 3 nucleotides at each position.
It was already known that DNA was composed of a sugar phosphate backbone, so all of these things taken together led Pauling to a triple helix.
In the figure above, Pauling and Robert Corey placed the phosphate backbones of 3 DNA strands at the center of the structure with the nucleotides facing out, like the spokes of a bicycle wheel.
In hindsight, we know this is totally wrong and there are 3 key errors that make this structure implausible:
1. At pH 7, the phosphate backbone is negatively charged so 3 of them packed together would repel one another
2. The model doesn't leave space for sodium and Astbury's diffractions were all sodium salts
3. Chargaff's nucleotide pairing rules were totally ignored
Fortunately, taking inspiration from Pauling and his use of modeling, Watson and Crick, armed with pristine x-ray diffraction data from Franklin and Gosling, published the correct double helical structure for DNA two months later in the April 1953 issue of Nature.
###
Pauling L, Corey RB. 1953. A Proposed Structure For The Nucleic Acids. PNAS. DOI: 10.1073/pnas.39.2.84
Were you forwarded this newsletter?
LOVE IT.
If you liked what you read, consider signing up for your own subscription here: