Omic.ly Weekly 44
October 6, 2024
Hey There!
Thanks for spending part of your Sunday with Omic.ly!
You may or may not have noticed you didn't receive an issue last Sunday.
That's because I was dealing with the fallout from Hurricane Helene.
Unfortunately, I live in the mountains of western North Carolina and some places saw up to 30" of rain over a 24hr period.
I lost power and internet for 5 days and will probably lose access to running water this weekend.
But we will persevere.
I've probably had it the easiest of anyone in the region.
My house didn't get washed away in flood waters, I've had food to eat, and we're well stocked with drinking water.
That is not true for many people here, and this last week has been very challenging for the local community.
But, I've had a few minutes this weekend to pick up where I left off before Helene dumped the Gulf of Mexico on Asheville.
Hopefully you have as much fun reading this issue as I did writing it.
It's been a nice break from the absolute devastation surrounding me.
Thanks for that.
And lucky for you, you can't smell me...
This Week's Headlines
1) Multi-omics can predict a thousand diseases now
2) Ethnic Stratification: Sounds complicated, but in genomics it helps everyone
3) Beighton and Astbury beat Gosling and Franklin to a pristine diffraction of DNA. They never shared it
Here's what you missed in this week's Premium Edition:
HOT TAKE: Let's Get Checked has bought TruePill to make at home healthcare a reality
Or if you already have a premium subscription:
MILTON is here to help predict 1,000 diseases before they happen
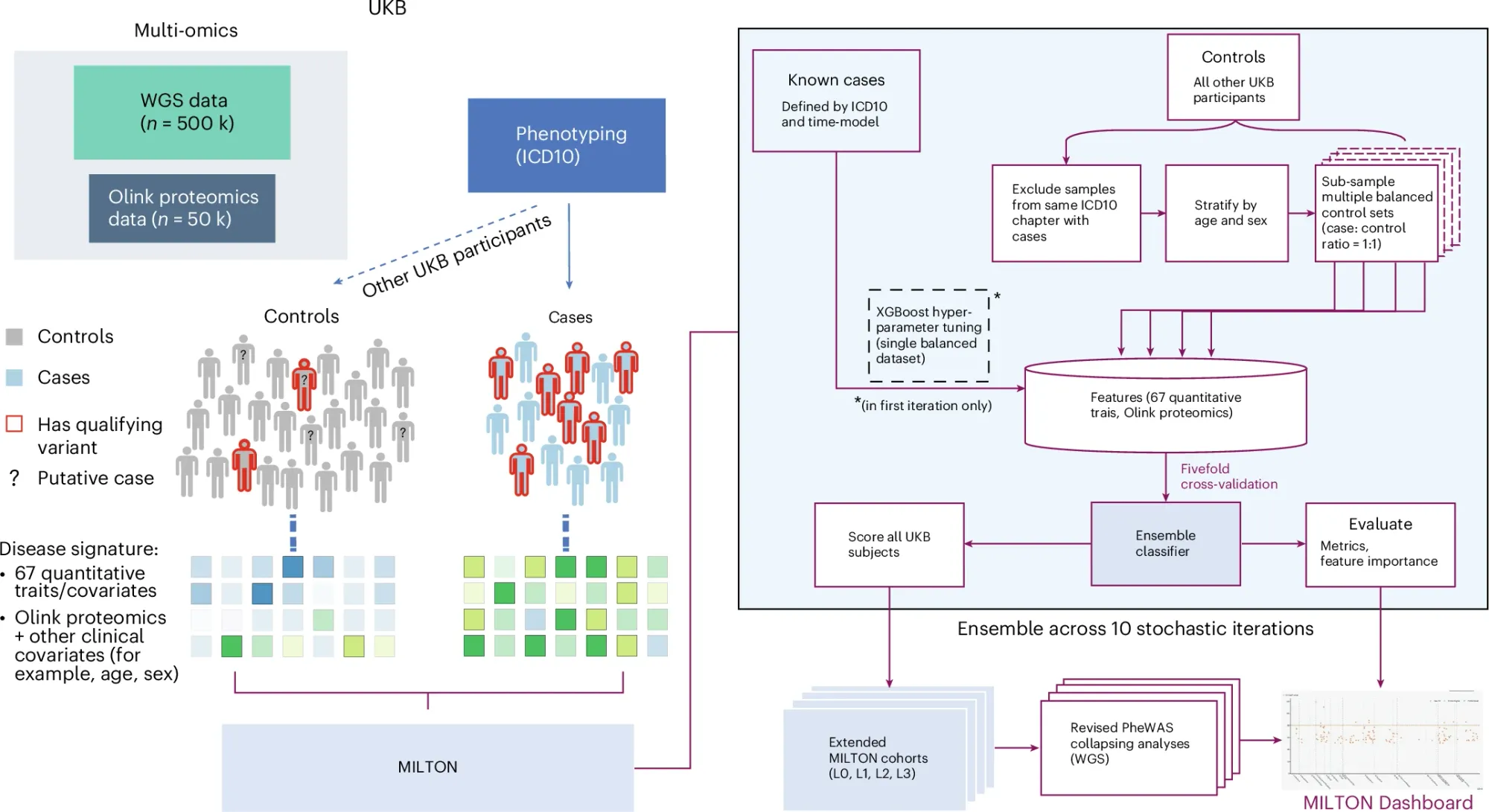
Have you ever wondered how we use all of the data generated in healthcare to predict and prevent disease before it occurs?
You're not alone, I wonder this a lot too...
Because, historically, we've been really bad at it!
Healthcare most places is setup to be reactive, to wait and see how symptoms develop, and to treat a disease once it is bad enough that we can name it.
But what if we could detect the signatures of a disease years or decades before onset and make proactive changes to better prepare for the development of disease in a patient, reduce symptom onset or even prevent the development of those diseases altogether?
That's been the promise of Precision Medicine for a long time, and, unfortunately, after over two decades of sequencing the human genome, we're still not at a place where we can broadly use 'omic tools to predict disease in the vast majority of people.
A big part of this is just that predicting disease in healthy populations is an enormously challenging problem to solve!
But another big piece here is we haven't had the right tools to generate the data we need to identify disease informative biomarkers.
We've certainly had sequencing for a while, but the sequence of our genomes isn't a great predictor of whether we'll actually develop a disease.
It represents potential, but it's hard to know 'how, when or if' potential problems in our genomes develop into a disease without looking at other things like proteins, metabolites, or other chemical signals that are generated by our cells.
These other things tell us what our bodies are actively doing!
And when analyzed in concert with genetic data, they can provide clues to when our genomes start misbehaving, sometimes a decade or more before symptom onset!
This has been shown to be true recently for a number of specific diseases, like the development of dementia, but a recent paper in Nature Genetics used genetic, proteomic, health record, and blood chemistry data from the UK Biobank to develop an algorithm to predict the onset of over 1,000 diseases.
The pipeline for this (Machine learning with phenotype associations, MILTON) can be seen in the figure above where data from cases and controls is used to identify novel disease predictive signatures.
MILTON had high predictive power for many diseases (AUCs greater than 0.7 for over 1,000 ICD10 codes, and AUCs over 0.9 for 121 codes).
It also outperformed gene specific polygenic risk scores for most diseases, but notable exceptions included conditions like melanoma, breast cancer, prostate cancer, and glaucoma.
While still early, and certainly not perfect, this work highlights the importance of integrating multi-omic and other biomarker data into the development and refinement of disease prediction models.
###
Garg M, et al. 2024. Disease prediction with multi-omics and biomarkers empowers case–control genetic discoveries in the UK Biobank. Nature Genetics. DOI: 10.1038/s41588-024-01898-1
Ethnic stratification and why single reference based analysis methods aren't 'good enough.'
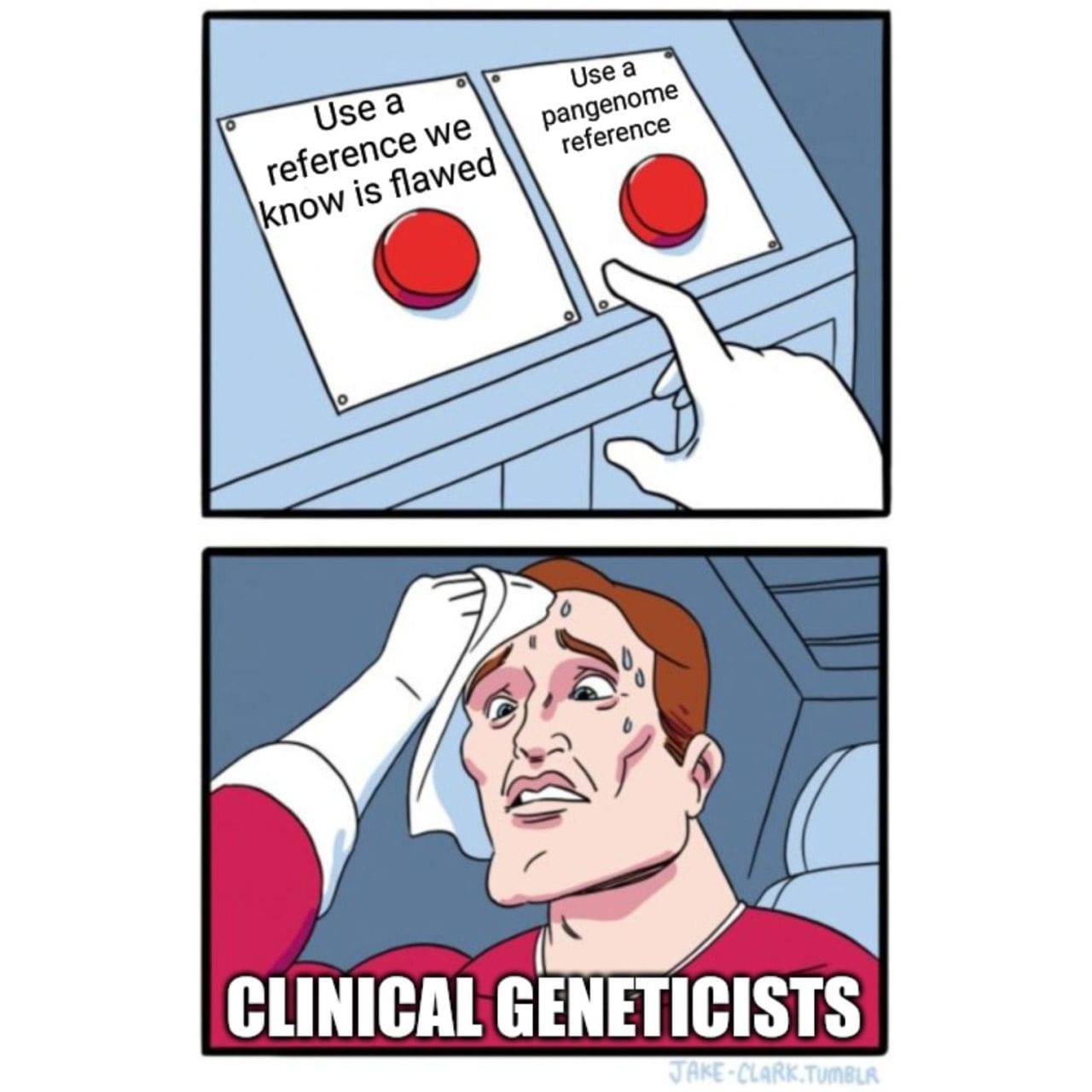
If you've done genetics for any amount of time you know that stratification of populations is important for getting useful information out of them.
But if you're not a geneticist, it might be a good idea to explain why this is true.
Stratification is a statistical term that basically means "divide your data into subgroups."
In genetics, we usually start with age, gender, life style/exposure, and ethnicity.
The reason for doing this is to be able to determine if subpopulations within a dataset are more or less likely to have whatever it is that you're looking for.
This is usually a measurable trait.
Sometimes this is a common trait like height or eye color, but in healthcare, we're usually talking about disease traits.
So, figuring out if a specific gender, age group, or ethnicity is predisposed to a disease is important, but because diverse populations have mostly been absent from clinical studies, it’s hard to identify important markers of disease in them.
While we know ‘variants’ or ‘mutations’ can contribute to disease, how these contribute to disease can differ vastly depending on someone’s ethnic background.
Variants in one ethnicity may not matter in another ethnicity because mutations elsewhere can compensate in some way for those changes.
So, how you go about determining what is or is not a variant can have a serious impact on the conclusions you draw from a dataset.
As it stands now, most variants are determined by comparing a patient’s genetic sequence to a ‘reference.’
The reference here is the one determined by the human genome project.
This was supposed to represent the sequence of the average healthy human, but we know now that the bulk of this DNA was provided by a mixed race male.
So ‘variations’ from this reference might not be super accurate if we’re trying to determine the importance of a variant in a different ethnicity.
This bears out in multiple clinical evaluations with a recent survey determining that the number of variants of unknown significance (VUS) was markedly higher in Africans (45%) than Caucasians (32%).
A good chunk of the VUS-ness here has to do with whether the ‘reference’ was appropriate for an African vs a Caucasian, but it also has a lot to do with the fact that most genetic studies have been done in Caucasians, so we already have an idea which ‘variants’ are significant in that population.
Fortunately, we’re seeing progress on multiple fronts here with most associations and government institutions calling for greater diversity in clinical trials.
We also have a human pangenome reference now which more accurately characterizes the ethnic differences we see in our genomes. It’s not completely done yet, but pangenome based variant calling pipelines are available.
So the question is: how long will it take to integrate these updates into clinical practice?
Elwyn Beighton and William Astbury generated a nearly flawless diffraction of B-DNA in 1951, a full year ahead of Franklin and Gosling. They never shared or published it.
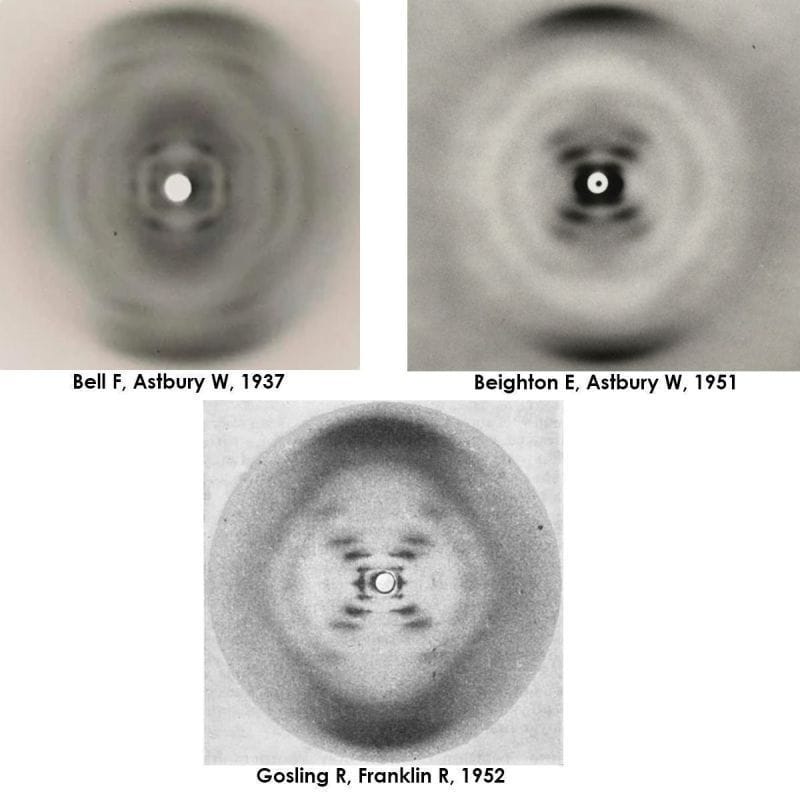
As with everything on the path to the discovery of the DNA double-helix, timing was everything!
Well, timing, and knowing what you're looking at.
William Astbury might not be a name you've heard before but he's considered a founder of molecular biology.
He was one of the first to use x-ray diffraction to study protein structures.
He was a protege of William Bragg who in 1915 won the Nobel Prize, along with his son Lawrence, for the discovery that x-rays could be used to determine the location of atoms within a molecule.
What the Bragg's found was that if you blasted crystals with x-rays, the x-rays bounced off of the atoms in those molecules to create specific patterns on x-ray film.
Working backwards, they realized they could deduce from those patterns the structure of the underlying molecule!
However, by 1926, Bragg got bored with blasting simple molecules and tasked his graduate student, Astbury, with studying larger fibrous biological molecules like wool.
Since textiles were an important commodity, studying their properties and how to modify them to improve their commercial value was a big deal.
This led Astbury to start a lab in 1928 in textile physics where he made a name for himself diffracting just about every biological fiber in existence.
So, it should be no surprise that in 1937 he turned his attention to the most important biological fiber of all, DNA.
He, along with a talented graduate student, Florence Bell, created the first ever diffractions for DNA and they published the first proposed structure for DNA, what they referred to as a 'pile of pennies,' in 1938.
Unfortunately, Bell and Astbury's work on DNA was cut short by World War II and Astbury didn't return to the problem of DNA until the late 1940's.
But by 1951, Elwyn Beighton, a lab tech turned graduate student, had picked up where Bell left off and produced a nearly perfect diffraction of DNA.
Unbeknownst to anyone at the time, DNA could take on two forms: a totally dehydrated A form, a hydrated B form, or a mix of both depending on the humidity during drying.
The figure above is actually 3 figures.
It has Bell's original diffraction of DNA (likely a mix of A and B), Beighton's significantly improved diffraction, and finally, photo 51, Franklin and Gosling's pristine diffraction of B-DNA.
All of the 'what ifs' aside, it's thought that Astbury was too preoccupied with protein structures to recognize the importance of Beighton's cruciform DNA diffraction.
The image was never published, and was lost to history.
Fortunately, the world only had to wait 2 additional years for the structure of DNA to be solved in 1953.
Were you forwarded this newsletter?
LOVE IT.
If you liked what you read, consider signing up for your own subscription here: