New tech always has risks and limitations, single-cell and spatial transcriptomics are no exception
Single-Cell and Spatial Transcriptomics: Risks, Limitations, and Questions to Ask
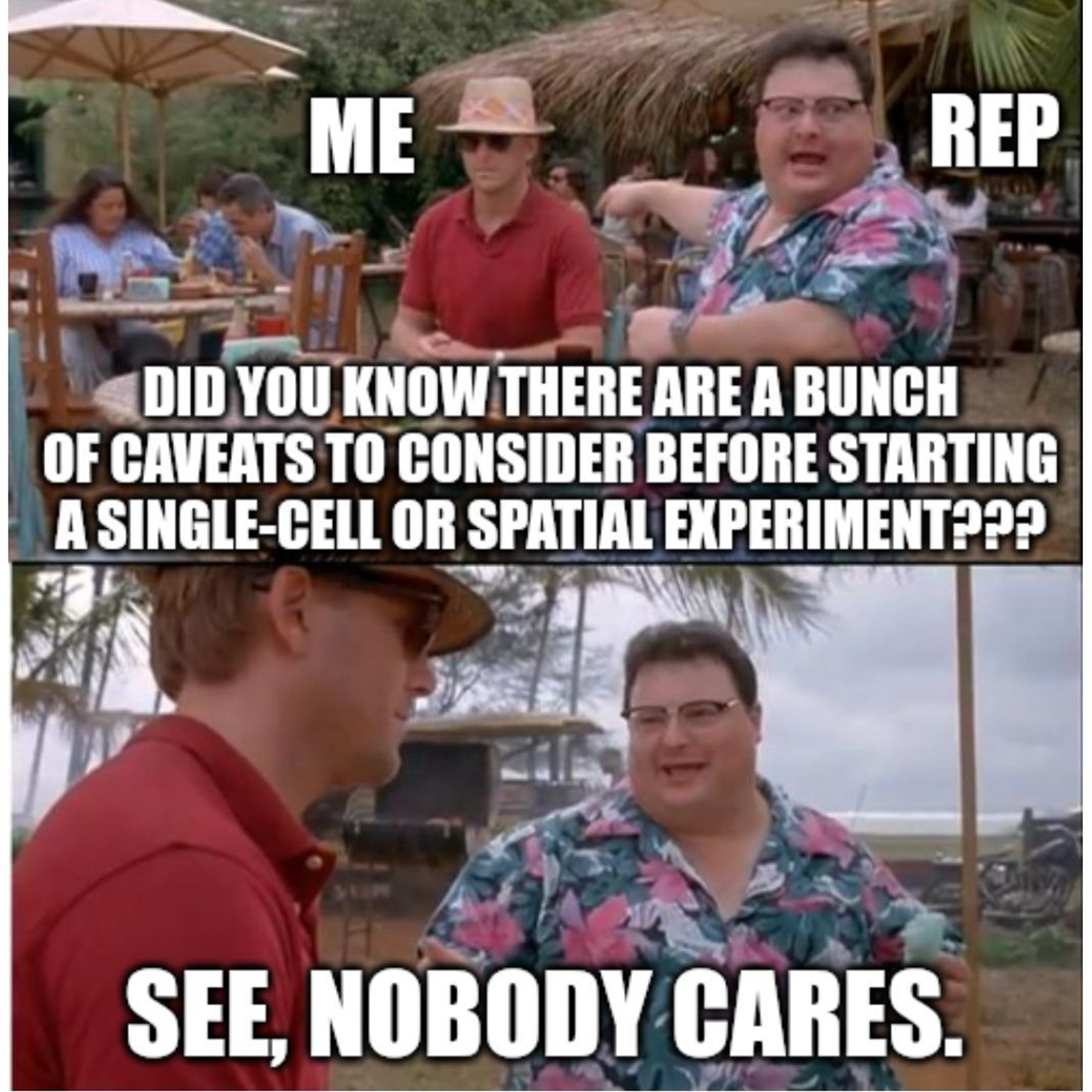
"Brian, why do you always have to be such a wet blanket?"
Ah, the age-old question...
But this wouldn't be science if we didn't also think about all the ways these things might not be telling us the whole story.
Or worse, telling us the wrong story!
Here are a few things to consider before you make a bunch of pretty pictures or tSNE plots:
Abundant Transcripts - Some RNAs are expressed much more than other RNAs. These are usually things like ‘housekeeping’ genes that are involved in supporting major cellular functions, for example, ribosomal RNA. These signals can dominate a dataset and hide information you care about seeing.
Sensitivity/Specificity - Do you pick up all the positive signals, are the negatives actually negative? Do you have enough reads/resolution to know? Controls are your friend here but not all controls are created equally! Is anyone working on synthetic single-cell controls (Are they??)?
Full-length vs 3'-UTRs/Hybridization Probes - Do you care about knowing what isoforms are present in your samples? Choosing the right methodology here is key because alternative splicing of transcripts can change what story you might tell if you only look at probe binding/sequencing from 3'-UTRs!
Recovery - This is single-cell specific, but the quality of your input cells and the technology you use can dramatically affect how many cells you actually get data from. Do your handling processes bias recovery? Do you have enough input to recover a representative population of cells?
Spatial Specific Limitations:
Resolution - Spatial resolutions are all over the place from 10um down to 100nm! That's a wide range and it means that in the lower resolution methods there's a chance you can get signal overlap and miss things that you might want to actually detect. Which leads to the next problem…
Fluorescence Interference/Crowding - Are you detecting the signals you think you're detecting or is there interference or crowding because two things you care about occupy the same space?
Transcript Accessibility - Are the RNAs you're trying to detect accessible for probe or sequencing primer binding? What if they're covered in proteins or twisted up? Does that mean you don't detect them!? The inside of a fixed cell is a messy place…
Quantifying Spatial Results - Some methods naturally capture results in a matrix or a grid which makes it slightly easier to know that your results are quantified properly but what about methods that claim cellular or subcellular localization? Can they actually accurately determine cell boundaries? Sometimes that answer is yes.
"So, Are you saying everything we're doing is worthless?"
Nope, plus this is a rapidly evolving field that's improving every day!