MILTON is here to help predict 1,000 diseases before they happen
Multi-omics can predict diseases now, kinda
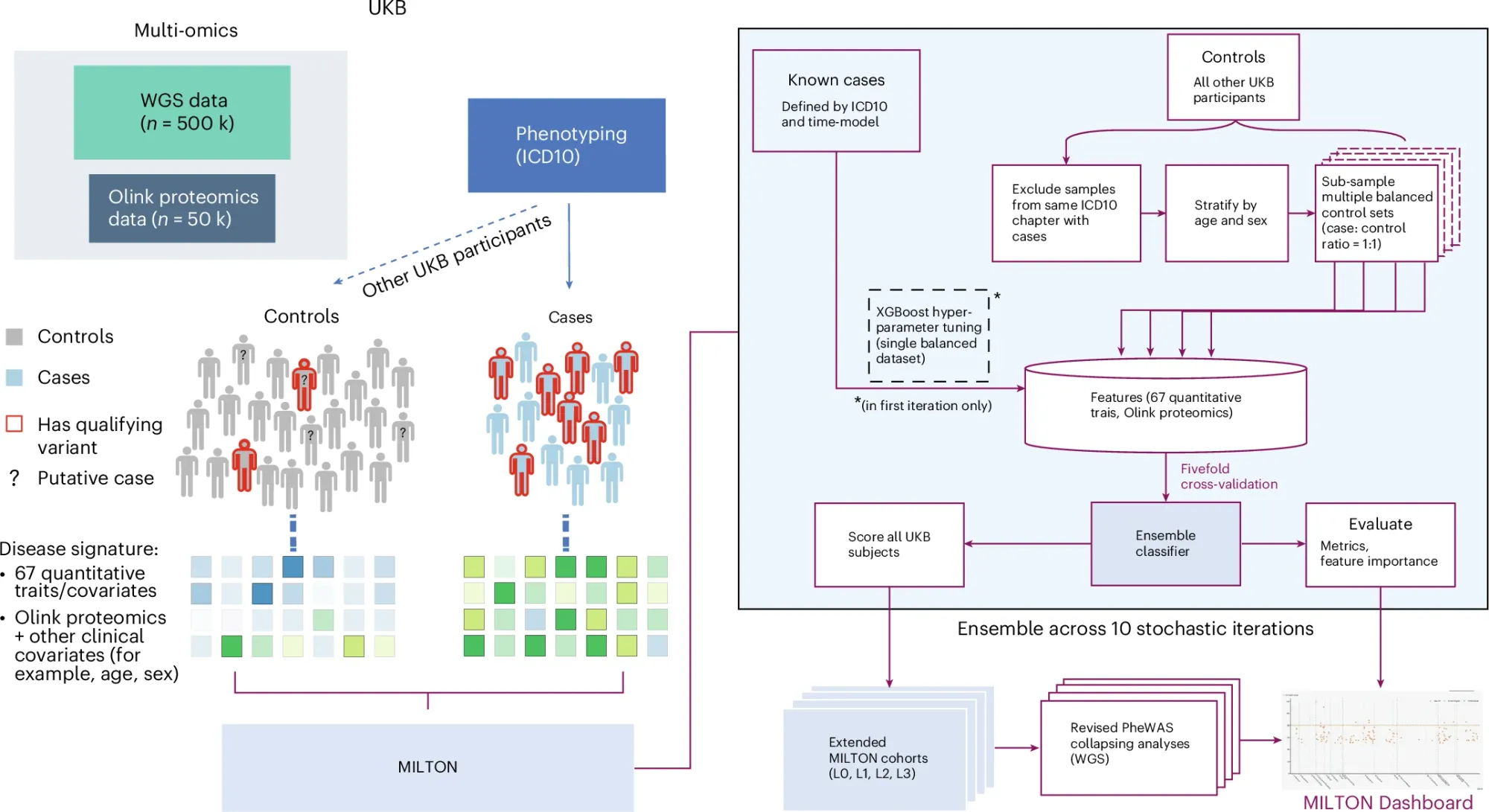
Have you ever wondered how we use all of the data generated in healthcare to predict and prevent disease before it occurs?
You're not alone, I wonder this a lot too...
Because, historically, we've been really bad at it!
Healthcare most places is setup to be reactive, to wait and see how symptoms develop, and to treat a disease once it is bad enough that we can name it.
But what if we could detect the signatures of a disease years or decades before onset and make proactive changes to better prepare for the development of disease in a patient, reduce symptom onset or even prevent the development of those diseases altogether?
That's been the promise of Precision Medicine for a long time, and, unfortunately, after over two decades of sequencing the human genome, we're still not at a place where we can broadly use 'omic tools to predict disease in the vast majority of people.
A big part of this is just that predicting disease in healthy populations is an enormously challenging problem to solve!
But another big piece here is we haven't had the right tools to generate the data we need to identify disease informative biomarkers.
We've certainly had sequencing for a while, but the sequence of our genomes isn't a great predictor of whether we'll actually develop a disease.
It represents potential, but it's hard to know 'how, when or if' potential problems in our genomes develop into a disease without looking at other things like proteins, metabolites, or other chemical signals that are generated by our cells.
These other things tell us what our bodies are actively doing!
And when analyzed in concert with genetic data, they can provide clues to when our genomes start misbehaving, sometimes a decade or more before symptom onset!
This has been shown to be true recently for a number of specific diseases, like the development of dementia, but a recent paper in Nature Genetics used genetic, proteomic, health record, and blood chemistry data from the UK Biobank to develop an algorithm to predict the onset of over 1,000 diseases.
The pipeline for this (Machine learning with phenotype associations, MILTON) can be seen in the figure above where data from cases and controls is used to identify novel disease predictive signatures.
MILTON had high predictive power for many diseases (AUCs greater than 0.7 for over 1,000 ICD10 codes, and AUCs over 0.9 for 121 codes).
It also outperformed gene specific polygenic risk scores for most diseases, but notable exceptions included conditions like melanoma, breast cancer, prostate cancer, and glaucoma.
While still early, and certainly not perfect, this work highlights the importance of integrating multi-omic and other biomarker data into the development and refinement of disease prediction models.