Foundation models are all the rage today - Now there's one for genomes!
EvoAI: a genome foundation model for building prokaryotes
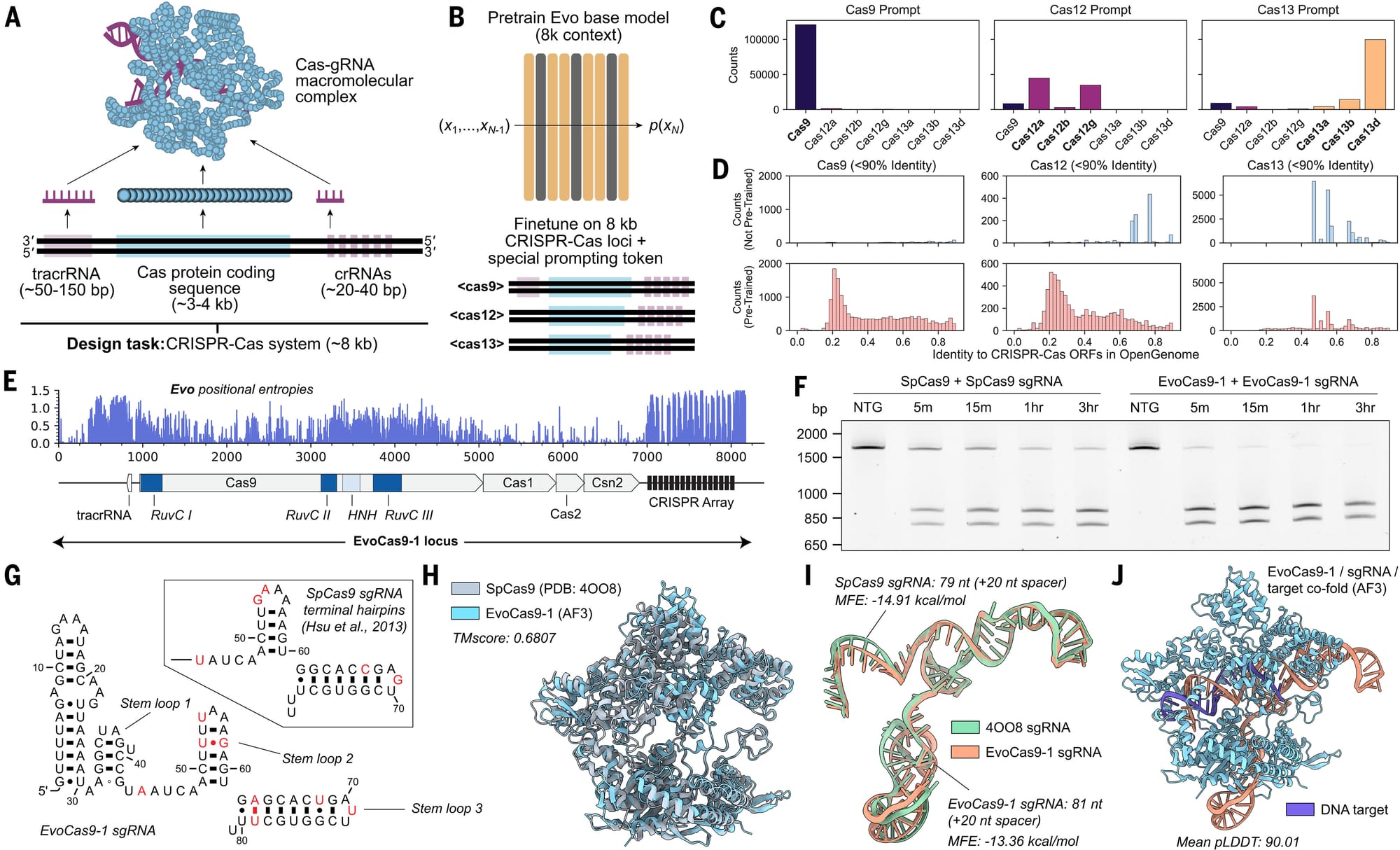
Generative AI models stormed into our lives just a few short years ago.
And they've allowed us to prompt engineer them to create new images or text.
For example, a user could ask OpenAI's DALL-E image generator to create a picture of salmon in a stream and it might sometimes create images of what appear to be live fish swimming in water.
Other times it spits out pictures of salmon fillets floating through those same scenes, but those kinds of issues just come with the tokenized statistical territory of generative AI models.
These models work by breaking down images or text into smaller more digestible units (tokens) to then learn how these components are all inter-related.
They can then use the statistics generated during that learning to create a new image or some text based on what they've seen before.
In the example above, a model trained on images of fish (the living kind and the sushi kind) could generate a wide variety of interesting images of "salmon" in a stream!
But the results of these models generally get better as you add context, so if you're really interested in seeing live fish swimming in a stream, be explicit!
"Brian, why did you just go on some weird tangent about AI model hallucinations?"
Because, there's a new foundation model for prokaryotic genomes and it too suffers from odd hallucinations!
But, if you understand how these models work, it is possible to prompt them to give you pictures of swimming fish, or in the case of a genome model, novel and functional protein complexes!
In this week's paper, researchers at Stanford's Arc Institute developed Evo, a foundation model "trained on 2.7 million raw prokaryotic (bacteria) and phage genome sequences."
The exact details of how the model was trained are a bit too math-y for my simple brain, but what makes this model unique is that it was trained at a single-nucleotide resolution but with a longer context length (131,000 base pairs).
The authors say this makes it better at predicting how things interact at a distance, which is important when you're developing a genome foundation model because lots of regulatory sequences act at a distance.
After some in silico benchmarking of their model they then got the genius idea to see if they could prompt it to generate new CRISPR proteins and guide RNAs.
But because they didn't want their model to spit out "fillets" when they were looking for "live fish," they retrained it around the context of CRISPR genes (about 8kb) and the results of that experiment can be seen in the figure above:
a) Overview of a CRISPR protein-RNA complex b) Overview of model retraining on an 8kb CRISPR specific context c) Shows that prompts mostly spit out what they're prompted to spit out! d) Pre-training (bottom) creates more structures than no pre-training (top) e) Positional entropy (randomness of sequence) for the best CRISPR-Cas the model generated, EvoCas9-1 f) Performance of the new CRISPR (right side - worked really well) to old CRISPR (left) g-j) Comparison of new CRISPR components to old CRISPR
The authors go on to state that "the EvoCas9-1 amino acid sequence shares 79.9% identity with the closest Cas9 in the database of Cas proteins used for model fine-tuning and 73.1% identity with SpCas9. Evo-designed sgRNA is 91.1% identical to the canonical SpCas9 sgRNA."
They also showed that they could use this model to generate novel transposons systems, and scale it up to create de novo prokaryotic genomes.
While they didn't validate the latter and said that these "genomes" definitely wouldn't be functional because they suffer from the same problems as other generative models ie hallucinations, refinements in the future could allow Evo to scale to Eukaryotic (multicellular) organisms.